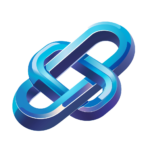
AI Driven Predictive Maintenance Scheduling Workflow Explained
Discover how AI-driven predictive maintenance scheduling enhances operational efficiency through data collection analysis and continuous improvement strategies
Category: AI Content Tools
Industry: Telecommunications
Predictive Maintenance Scheduling
1. Data Collection
1.1 Identify Data Sources
Gather data from various sources including network performance metrics, equipment logs, and customer feedback.
1.2 Utilize AI-Driven Tools
Implement tools such as IBM Watson IoT and Microsoft Azure IoT for real-time data acquisition and analysis.
2. Data Analysis
2.1 Data Processing
Use AI algorithms to clean and preprocess the collected data to ensure accuracy and relevance.
2.2 Predictive Analytics
Employ machine learning models such as TensorFlow and Apache Spark to analyze historical data and identify patterns that predict potential equipment failures.
3. Maintenance Scheduling
3.1 Develop Maintenance Models
Create predictive maintenance models that determine optimal maintenance schedules based on the analysis.
3.2 Integration with Scheduling Tools
Integrate AI-driven scheduling tools like ServiceMax or UpKeep to automate and optimize the maintenance workflow.
4. Implementation
4.1 Execute Maintenance Tasks
Deploy maintenance teams based on the predictive insights provided by the AI models.
4.2 Monitor Outcomes
Utilize monitoring tools such as Splunk to track the effectiveness of maintenance actions and adjust strategies as necessary.
5. Continuous Improvement
5.1 Feedback Loop
Establish a feedback loop that incorporates outcomes back into the data collection phase for continuous learning and improvement.
5.2 Update AI Models
Regularly update machine learning models with new data to enhance predictive accuracy using tools like Google Cloud AI.
Keyword: predictive maintenance scheduling tools