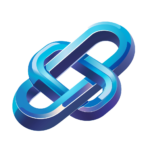
AI Driven Predictive Maintenance Workflow for Kitchen Equipment
AI-driven predictive maintenance for kitchen equipment enhances food waste management through real-time monitoring data analysis and proactive scheduling
Category: AI Cooking Tools
Industry: Food Waste Management
Predictive Maintenance for Kitchen Equipment
Overview
This workflow outlines the process of implementing predictive maintenance for kitchen equipment using AI cooking tools to enhance food waste management. The integration of artificial intelligence allows for real-time monitoring, data analysis, and proactive maintenance scheduling.
Workflow Steps
1. Initial Assessment
Conduct an assessment of current kitchen equipment and identify key areas for improvement related to maintenance and food waste management.
2. Data Collection
Utilize IoT sensors and devices to collect data from kitchen equipment, including:
- Temperature readings
- Operational hours
- Usage patterns
- Failure rates
3. AI Integration
Implement AI-driven tools to analyze collected data. Examples include:
- IBM Watson IoT: Leverage this platform for real-time analytics and predictive insights.
- Uptake: Utilize their AI solutions for predictive analytics specific to kitchen equipment.
- Google Cloud AI: Use machine learning models to predict equipment failures and optimize maintenance schedules.
4. Predictive Analytics
Analyze the data using AI algorithms to forecast potential equipment failures and maintenance needs. This step includes:
- Identifying patterns in equipment performance
- Estimating the remaining useful life of equipment
- Generating predictive maintenance schedules
5. Maintenance Scheduling
Based on predictive analytics, schedule maintenance activities to minimize downtime and prevent food waste. This involves:
- Creating a maintenance calendar
- Notifying staff of upcoming maintenance tasks
- Documenting completed maintenance activities
6. Continuous Monitoring
Establish a continuous monitoring system to ensure ongoing data collection and analysis. This includes:
- Regular updates to AI algorithms based on new data
- Adjusting maintenance schedules as needed
- Monitoring food waste metrics to evaluate the effectiveness of predictive maintenance
7. Reporting and Feedback
Generate reports on maintenance activities, equipment performance, and food waste reduction. Solicit feedback from kitchen staff to improve the workflow.
8. Iteration and Improvement
Continuously refine the predictive maintenance process based on feedback and evolving AI technologies. This ensures the workflow remains effective and aligned with food waste management goals.
Conclusion
By implementing a predictive maintenance workflow for kitchen equipment using AI tools, organizations can significantly reduce food waste, enhance operational efficiency, and improve overall kitchen performance.
Keyword: Predictive maintenance kitchen equipment