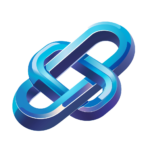
AI Driven Customer Churn Prediction and Retention Strategies
AI-driven customer churn prediction enhances retention by analyzing data and implementing targeted strategies for at-risk customers and continuous improvement
Category: AI Customer Service Tools
Industry: Telecommunications
Customer Churn Prediction and Retention
1. Data Collection
1.1 Identify Data Sources
Gather data from various sources including:
- Customer interactions (calls, chats, emails)
- Billing and payment history
- Customer feedback and surveys
- Social media interactions
1.2 Implement AI-Driven Tools
Utilize AI tools such as:
- Google Cloud AI: For natural language processing to analyze customer interactions.
- IBM Watson: To gather insights from unstructured data.
2. Data Preprocessing
2.1 Data Cleaning
Remove duplicates and irrelevant information to ensure data quality.
2.2 Feature Engineering
Identify key features that influence churn, such as:
- Usage patterns
- Customer satisfaction scores
- Payment timeliness
3. Churn Prediction Model Development
3.1 Select Machine Learning Algorithms
Choose appropriate algorithms for prediction, including:
- Random Forest
- Gradient Boosting Machines
- Neural Networks
3.2 Model Training
Train the model using historical data to predict churn likelihood.
3.3 Model Validation
Validate the model using techniques such as:
- Cross-validation
- Confusion matrix analysis
4. Implementation of Retention Strategies
4.1 Identify At-Risk Customers
Utilize the churn prediction model to flag customers with high churn risk.
4.2 Develop Targeted Retention Campaigns
Create personalized retention strategies using:
- Incentives (discounts, loyalty programs)
- Proactive customer service outreach
- Customized communication based on customer preferences
5. Monitoring and Optimization
5.1 Track Campaign Performance
Use analytics tools to measure the effectiveness of retention efforts.
5.2 Continuous Improvement
Leverage AI tools such as:
- Tableau: For data visualization and performance tracking.
- Salesforce Einstein: To refine predictive models based on new data.
6. Feedback Loop
6.1 Gather Customer Feedback
Collect ongoing feedback from retained customers to assess satisfaction and areas for improvement.
6.2 Update Models and Strategies
Regularly update the prediction models and retention strategies based on feedback and changing customer behaviors.
Keyword: Customer churn prediction strategies