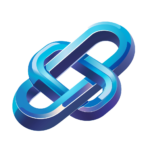
AI-Driven Personalized Medicine Development Workflow Guide
Discover an AI-driven personalized medicine development workflow that enhances patient care through data collection analysis drug discovery and continuous improvement
Category: AI Data Tools
Industry: Pharmaceuticals
Personalized Medicine Development Workflow
1. Research and Data Collection
1.1 Identify Target Diseases
Utilize AI-driven data mining tools such as IBM Watson to analyze medical literature and identify prevalent diseases suitable for personalized medicine approaches.
1.2 Collect Patient Data
Gather patient genomic, phenotypic, and clinical data using platforms like 23andMe and Illumina. Ensure compliance with data privacy regulations.
2. Data Integration and Preprocessing
2.1 Data Cleaning
Employ tools like OpenRefine to clean and standardize collected data, ensuring accuracy for further analysis.
2.2 Data Integration
Use ETL (Extract, Transform, Load) tools such as Talend to integrate disparate data sources into a cohesive database.
3. AI-Driven Data Analysis
3.1 Genomic Data Analysis
Implement AI algorithms using platforms like DeepVariant to analyze genomic sequences and identify variations associated with specific diseases.
3.2 Predictive Modeling
Utilize machine learning tools such as TensorFlow to develop predictive models that can anticipate patient responses to various treatments based on their genetic makeup.
4. Drug Discovery and Development
4.1 Target Identification
Leverage AI tools like Atomwise to screen compounds and identify potential drug targets based on biological data.
4.2 Preclinical Testing
Utilize simulation software such as Simcyp to model drug interactions and predict pharmacokinetics in virtual patient populations.
5. Clinical Trials
5.1 Patient Stratification
Employ AI-driven analytics platforms like Medidata to stratify patients based on genetic profiles, ensuring appropriate trial participant selection.
5.2 Monitoring and Data Analysis
Use real-time data analytics tools like Oracle’s Siebel CTMS to monitor trial progress and analyze outcomes efficiently.
6. Regulatory Submission and Approval
6.1 Compile Dossier
Utilize AI tools for document management such as Veeva Vault to compile and submit regulatory documents to health authorities.
6.2 Post-Market Surveillance
Implement AI-driven pharmacovigilance systems like Bioclinica to monitor drug safety and efficacy post-approval, ensuring ongoing compliance and patient safety.
7. Continuous Improvement and Feedback Loop
7.1 Data Feedback
Use AI analytics to gather feedback from clinical outcomes and patient experiences to refine treatment protocols and drug formulations.
7.2 Iterative Development
Regularly update AI models with new data to enhance predictive accuracy and adapt to emerging trends in personalized medicine.
Keyword: personalized medicine development workflow