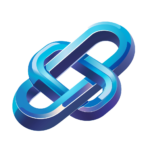
AI Driven Precision Crop Monitoring and Management Workflow
AI-driven precision crop monitoring enhances agricultural efficiency through data collection analysis and real-time decision-making for optimal yields
Category: AI Data Tools
Industry: Agriculture
Precision Crop Monitoring and Management
1. Data Collection
1.1 Soil Analysis
Utilize soil sensors to collect data on moisture, pH levels, and nutrient content.
1.2 Weather Monitoring
Implement weather stations equipped with IoT sensors to gather real-time weather data.
1.3 Crop Health Imaging
Employ drones and satellite imagery to capture high-resolution images of crop health.
2. Data Processing
2.1 Data Integration
Aggregate data from various sources using platforms such as Microsoft Azure or Google Cloud.
2.2 Data Cleaning
Utilize AI algorithms to filter out noise and irrelevant data for accuracy.
3. Data Analysis
3.1 Predictive Analytics
Leverage AI tools like IBM Watson to analyze historical data and predict crop yields.
3.2 Anomaly Detection
Use machine learning models to identify abnormal patterns in crop growth or soil conditions.
4. Decision-Making
4.1 Actionable Insights
Generate reports with actionable insights using data visualization tools such as Tableau.
4.2 Resource Allocation
Implement AI-driven decision support systems to optimize resource allocation (water, fertilizers, etc.).
5. Implementation
5.1 Precision Agriculture Techniques
Utilize variable rate technology (VRT) for targeted application of inputs based on AI analysis.
5.2 Continuous Monitoring
Deploy AI-driven platforms like CropX for ongoing monitoring and adjustments in real-time.
6. Evaluation
6.1 Performance Metrics
Establish KPIs to measure crop yield, input efficiency, and cost-effectiveness.
6.2 Feedback Loop
Incorporate feedback mechanisms to refine AI models and improve future decision-making processes.
Keyword: AI crop monitoring solutions