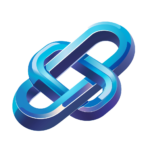
AI Integration in Population Health Management Workflow Guide
AI-driven population health management enhances care through data collection analysis risk stratification care coordination and outcome measurement for improved patient health
Category: AI Data Tools
Industry: Healthcare
AI-Driven Population Health Management
1. Data Collection
1.1 Identify Data Sources
- Electronic Health Records (EHRs)
- Wearable Health Devices
- Patient Surveys and Feedback
- Social Determinants of Health (SDOH) Data
1.2 Implement Data Integration Tools
- Use tools like Mirth Connect for interoperability.
- Leverage Apache NiFi for data flow automation.
2. Data Analysis
2.1 Data Cleaning and Preparation
- Utilize Trifacta for data wrangling and transformation.
- Implement Talend for data quality management.
2.2 Predictive Analytics
- Employ IBM Watson Health for predictive modeling.
- Utilize Google Cloud AI for machine learning algorithms.
3. Risk Stratification
3.1 Identify High-Risk Populations
- Use AI algorithms to analyze patient data and identify risk factors.
- Implement OptumIQ for risk stratification analytics.
3.2 Develop Targeted Interventions
- Create tailored care plans using insights from AI analysis.
- Utilize Health Catalyst for intervention tracking.
4. Care Coordination
4.1 Engage Care Teams
- Implement CareCloud for seamless communication among healthcare providers.
- Use Epic Systems for care management tools.
4.2 Monitor Patient Engagement
- Utilize MyChart for patient portal engagement tracking.
- Leverage WellDoc for real-time patient feedback.
5. Outcome Measurement
5.1 Evaluate Health Outcomes
- Use Tableau for data visualization and outcome reporting.
- Implement Qlik Sense for comprehensive analytics.
5.2 Continuous Improvement
- Establish a feedback loop using AI insights for ongoing program enhancement.
- Utilize Microsoft Power BI for tracking performance metrics.
Keyword: AI-driven population health management