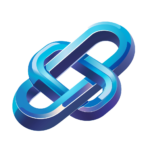
AI Integration in Student Support Workflow for Enhanced Learning
AI-driven student support enhances learning through data collection predictive analytics personalized plans continuous monitoring and ongoing evaluation for improved outcomes
Category: AI Data Tools
Industry: Education
AI-Driven Student Support and Intervention
1. Data Collection and Analysis
1.1 Identify Data Sources
- Student performance data (grades, attendance)
- Behavioral data (engagement in class, participation)
- Feedback from teachers and parents
1.2 Implement AI Data Tools
- Example Tools:
- Google Cloud AI for Education
- IBM Watson Education
- Microsoft Azure Machine Learning
2. Predictive Analytics
2.1 Utilize AI Algorithms
- Develop predictive models to identify at-risk students.
- Analyze trends in student performance over time.
2.2 Generate Insights
- Provide actionable insights for educators.
- Highlight areas needing intervention.
3. Personalized Learning Plans
3.1 Create Tailored Interventions
- Utilize AI to design individualized learning paths.
- Incorporate adaptive learning technologies.
3.2 Implement AI-Driven Tools
- Example Tools:
- Knewton
- DreamBox Learning
- Smart Sparrow
4. Continuous Monitoring and Feedback
4.1 Track Progress
- Use AI to continuously monitor student engagement and performance.
- Adjust learning plans based on real-time data.
4.2 Provide Feedback Loops
- Facilitate communication between students, teachers, and parents.
- Utilize AI chatbots for instant feedback and support.
5. Evaluation and Improvement
5.1 Assess Effectiveness
- Evaluate the impact of AI-driven interventions on student outcomes.
- Use data analytics to refine strategies.
5.2 Iterate on Processes
- Continuously improve AI models and tools based on feedback.
- Stay updated with the latest advancements in AI for education.
Keyword: AI driven student support system