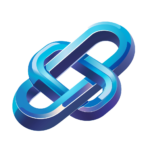
AI Powered Smart Harvesting and Yield Prediction Workflow
Discover an AI-driven workflow for smart harvesting and yield prediction that enhances farming efficiency through real-time data analysis and actionable insights.
Category: AI Data Tools
Industry: Agriculture
Smart Harvesting and Yield Prediction Workflow
1. Data Collection
1.1 Sensor Deployment
Utilize IoT sensors in fields to gather real-time data on soil moisture, temperature, and crop health.
1.2 Satellite Imagery
Implement satellite imaging technology to monitor crop growth and assess field conditions.
1.3 Historical Data Analysis
Collect historical yield data and weather patterns to establish a baseline for predictions.
2. Data Processing
2.1 Data Integration
Consolidate data from various sources (sensors, satellites, historical records) into a centralized database.
2.2 Data Cleaning
Utilize AI-driven tools such as DataRobot to clean and preprocess data for analysis.
3. AI Model Development
3.1 Machine Learning Algorithms
Develop machine learning models using platforms like TensorFlow or PyTorch to predict crop yield based on collected data.
3.2 Model Training
Train models using historical data to identify patterns and improve prediction accuracy.
4. Yield Prediction
4.1 Real-time Analysis
Employ AI tools such as IBM Watson to analyze current data and provide yield forecasts.
4.2 Scenario Simulation
Use simulation tools like Agri-Tech East to assess different farming scenarios and their potential impacts on yield.
5. Decision Support
5.1 Actionable Insights
Generate reports and visualizations using Tableau to present insights on expected yields and optimal harvesting times.
5.2 Recommendations
Provide farmers with AI-driven recommendations for resource allocation and harvesting strategies.
6. Implementation
6.1 Smart Harvesting Techniques
Integrate autonomous harvesting equipment that utilizes AI for efficient crop collection.
6.2 Continuous Monitoring
Set up ongoing monitoring systems to adjust strategies based on real-time data and predictions.
7. Feedback Loop
7.1 Performance Evaluation
Analyze the effectiveness of yield predictions against actual outcomes to refine AI models.
7.2 Iterative Improvement
Continuously update data inputs and models to enhance prediction accuracy and farming practices.
Keyword: AI driven crop yield prediction