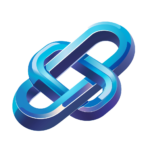
Automated Clinical Trial Design with AI Integration Workflow
AI-driven clinical trial design optimizes planning patient recruitment data management and regulatory submission enhancing overall trial efficiency and outcomes
Category: AI Data Tools
Industry: Pharmaceuticals
Automated Clinical Trial Design and Optimization
1. Initial Planning and Feasibility Assessment
1.1 Define Objectives
Establish the primary goals of the clinical trial, including endpoints and target populations.
1.2 Feasibility Study
Utilize AI-driven analytics tools such as IBM Watson for Clinical Trial Matching to assess the feasibility of the trial based on historical data and patient availability.
1.3 Stakeholder Engagement
Engage with stakeholders, including regulatory bodies and patient advocacy groups, to gather insights and align objectives.
2. Protocol Development
2.1 Design Protocol
Draft the clinical trial protocol, incorporating AI insights to optimize study design and patient recruitment strategies.
2.2 Risk Assessment
Implement AI tools like Medidata’s AI Risk Assessment to identify potential risks and mitigation strategies.
3. Patient Recruitment and Retention
3.1 Identify Target Population
Use AI algorithms to analyze electronic health records (EHRs) and identify eligible patients.
3.2 Recruitment Strategies
Employ AI-powered platforms such as TrialX to enhance outreach and engagement with potential participants.
3.3 Retention Monitoring
Utilize predictive analytics tools to monitor patient engagement and implement retention strategies proactively.
4. Data Collection and Management
4.1 Electronic Data Capture (EDC)
Implement EDC systems like Medidata Rave for streamlined data collection and real-time monitoring.
4.2 AI-Driven Data Validation
Utilize AI tools to automate data cleaning and validation, ensuring high-quality datasets for analysis.
5. Data Analysis and Interpretation
5.1 Statistical Analysis
Apply AI-based statistical analysis tools such as SAS Viya to conduct complex analyses efficiently.
5.2 Predictive Modeling
Leverage machine learning algorithms to predict outcomes and optimize trial parameters based on interim data.
6. Reporting and Regulatory Submission
6.1 Generate Reports
Automate the generation of clinical study reports using AI-driven documentation tools.
6.2 Regulatory Submission
Prepare and submit regulatory documents utilizing platforms like Veeva Vault for compliance and tracking.
7. Post-Trial Analysis and Feedback
7.1 Analyze Outcomes
Conduct a thorough analysis of trial outcomes using AI tools to derive insights and lessons learned.
7.2 Stakeholder Feedback
Gather feedback from stakeholders and participants to improve future trial designs.
8. Continuous Improvement
8.1 Update Protocols
Utilize insights gained from AI analyses to refine and enhance clinical trial protocols for future studies.
8.2 AI Model Refinement
Continuously improve AI models based on new data and outcomes to enhance predictive capabilities for future trials.
Keyword: automated clinical trial optimization