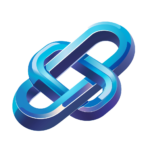
Automated A/B Testing with AI for Enhanced Conversion Rates
AI-driven automated A/B testing enhances conversion rates by defining objectives segmenting audiences and analyzing results for continuous improvement
Category: AI Developer Tools
Industry: Marketing and Advertising
Automated A/B Testing and Conversion Rate Optimization
1. Define Objectives and Key Performance Indicators (KPIs)
1.1 Identify Goals
Establish clear objectives for the A/B testing campaign, such as increasing click-through rates, improving conversion rates, or enhancing user engagement.
1.2 Determine KPIs
Specify measurable KPIs that align with the goals, such as number of sign-ups, sales conversions, or bounce rates.
2. Audience Segmentation
2.1 Analyze User Data
Utilize AI-driven analytics tools like Google Analytics or Mixpanel to gather insights on user behavior and demographics.
2.2 Segment Audience
Leverage machine learning algorithms to create specific audience segments based on behavior, preferences, and historical data.
3. Hypothesis Development
3.1 Generate Hypotheses
Use AI tools such as Optimizely or VWO to analyze existing data and generate hypotheses for potential improvements.
3.2 Prioritize Hypotheses
Evaluate the potential impact and feasibility of each hypothesis using predictive analytics.
4. Design A/B Tests
4.1 Create Variations
Develop different versions of the marketing asset (e.g., landing pages, emails) using design tools like Canva or Adobe XD.
4.2 Implement AI-Driven Personalization
Utilize AI solutions such as Dynamic Yield or Evergage to personalize content dynamically based on user segments.
5. Execute A/B Tests
5.1 Launch Tests
Deploy A/B tests using platforms like Google Optimize or Unbounce, ensuring that traffic is evenly distributed across variations.
5.2 Monitor Performance
Utilize real-time analytics tools to track performance metrics and user interactions during the testing phase.
6. Analyze Results
6.1 Collect Data
Gather data on user interactions and conversion rates for each variation using AI analytics tools.
6.2 Evaluate Outcomes
Apply statistical analysis to determine the significance of results, using tools like R or Python for advanced analysis.
7. Implement Findings
7.1 Optimize Based on Results
Choose the winning variation and implement changes across all relevant platforms.
7.2 Continuous Improvement
Establish a feedback loop utilizing AI to continually learn from user interactions and refine future A/B tests.
8. Report and Document
8.1 Create a Comprehensive Report
Document the A/B testing process, results, and insights gained, using tools like Tableau for data visualization.
8.2 Share with Stakeholders
Present findings to stakeholders to align on future marketing strategies and optimization efforts.
Keyword: AI driven A/B testing strategies