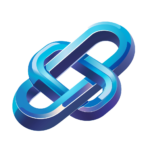
Optimize Drug Discovery Pipeline with AI Integration Solutions
AI-driven drug discovery pipeline optimizes target identification hit identification and clinical trials enhancing efficiency and accelerating therapy development
Category: AI Developer Tools
Industry: Pharmaceuticals and Biotechnology
Drug Discovery Pipeline Optimization
1. Target Identification
Utilize AI algorithms to analyze biological data and identify potential drug targets.
- Tools:
- DeepMind’s AlphaFold for protein structure prediction
- IBM Watson for Drug Discovery to identify novel targets
2. Hit Identification
Implement machine learning models to screen large compound libraries for potential hits.
- Tools:
- Atomwise for virtual screening using deep learning
- Schrödinger’s Glide for docking simulations
3. Hit-to-Lead Optimization
Employ AI-driven predictive analytics to optimize lead compounds for efficacy and safety.
- Tools:
- ChemAxon for cheminformatics and property prediction
- Insilico Medicine for generative drug design
4. Preclinical Development
Use AI to predict pharmacokinetics and toxicity profiles of lead candidates.
- Tools:
- ADMET Predictor for absorption, distribution, metabolism, excretion, and toxicity predictions
- DeepChem for molecular property predictions
5. Clinical Trials Design
Leverage AI to optimize trial design, patient recruitment, and data analysis.
- Tools:
- TrialStat for trial management and data collection
- Medidata for patient recruitment analytics
6. Post-Market Surveillance
Implement AI systems for real-time monitoring of drug performance and adverse effects.
- Tools:
- Oracle’s Argus for pharmacovigilance
- IBM Watson for analyzing real-world data and patient outcomes
Conclusion
By integrating AI-driven tools throughout the drug discovery pipeline, pharmaceutical and biotechnology companies can enhance efficiency, reduce costs, and accelerate the development of innovative therapies.
Keyword: AI drug discovery optimization