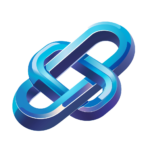
AI Driven Predictive Demand Forecasting Workflow for Businesses
AI-driven predictive demand forecasting workflow enhances accuracy through data collection model training and continuous monitoring for optimized decision making
Category: AI Finance Tools
Industry: Hospitality and Tourism
Predictive Demand Forecasting Workflow
1. Data Collection
1.1 Historical Data
Gather historical data on occupancy rates, booking patterns, customer demographics, and seasonal trends.
1.2 External Data Sources
Integrate external data sources such as local events, economic indicators, and weather forecasts to enhance the dataset.
2. Data Preparation
2.1 Data Cleaning
Utilize AI-driven tools like Trifacta or Talend to clean and preprocess the data, ensuring accuracy and consistency.
2.2 Data Enrichment
Enhance the dataset by incorporating additional variables that may impact demand, such as competitor pricing and marketing activities.
3. Model Selection
3.1 AI Model Identification
Select appropriate AI models for demand forecasting, such as ARIMA, Random Forest, or Neural Networks.
3.2 Tool Selection
Utilize AI finance tools like IBM Watson Studio or Google Cloud AI for model development and training.
4. Model Training and Validation
4.1 Training the Model
Use historical data to train the selected AI models, allowing them to learn patterns and relationships within the data.
4.2 Validation
Validate the model’s accuracy using a subset of data, employing metrics such as Mean Absolute Error (MAE) or Root Mean Squared Error (RMSE).
5. Forecast Generation
5.1 Demand Forecasting
Generate demand forecasts using the validated models, providing insights on expected occupancy rates and customer bookings.
5.2 Visualization
Utilize visualization tools like Tableau or Power BI to present forecasts in an understandable format for stakeholders.
6. Implementation and Monitoring
6.1 Strategy Development
Develop strategies based on demand forecasts to optimize pricing, staffing, and inventory management.
6.2 Continuous Monitoring
Implement AI tools such as Salesforce Einstein to continuously monitor demand fluctuations and adjust forecasts accordingly.
7. Feedback Loop
7.1 Performance Review
Regularly review forecast performance against actual outcomes to identify areas for improvement.
7.2 Model Refinement
Refine and retrain models based on feedback and new data to enhance forecasting accuracy over time.
Keyword: AI predictive demand forecasting