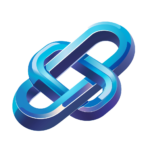
AI Driven Predictive Enrollment and Revenue Forecasting Workflow
AI-driven workflow for predictive enrollment and revenue forecasting enhances decision-making through data collection modeling and continuous improvement
Category: AI Finance Tools
Industry: Education
Predictive Enrollment and Revenue Forecasting
1. Data Collection
1.1 Identify Data Sources
Gather historical enrollment data, financial records, demographic information, and market trends.
1.2 Data Integration
Utilize AI-driven data integration tools such as Talend or Apache NiFi to consolidate data from various sources into a central repository.
2. Data Cleaning and Preparation
2.1 Data Validation
Ensure data accuracy by employing AI algorithms to identify and rectify inconsistencies.
2.2 Data Transformation
Use tools like Alteryx to transform raw data into a structured format suitable for analysis.
3. Predictive Modeling
3.1 Select AI Models
Choose appropriate predictive modeling techniques such as regression analysis, decision trees, or neural networks.
3.2 Implement AI Tools
Utilize platforms like IBM Watson Studio or Google Cloud AI to build and train predictive models based on historical data.
4. Forecasting Enrollment
4.1 Generate Enrollment Predictions
Leverage the trained AI models to forecast future enrollment numbers based on various scenarios.
4.2 Analyze Influencing Factors
Identify key factors affecting enrollment, such as economic indicators and demographic shifts, using tools like Tableau for visualization.
5. Revenue Forecasting
5.1 Calculate Revenue Projections
Integrate enrollment forecasts with tuition rates and funding sources to estimate future revenue.
5.2 Scenario Analysis
Conduct scenario analyses using AI simulations to assess the impact of different enrollment levels on revenue.
6. Reporting and Visualization
6.1 Develop Dashboards
Create interactive dashboards using Power BI or Google Data Studio to present forecasts to stakeholders.
6.2 Share Insights
Provide regular updates and insights to decision-makers to inform strategic planning.
7. Continuous Improvement
7.1 Monitor Outcomes
Track actual enrollment and revenue against forecasts to evaluate model accuracy.
7.2 Refine Models
Continuously enhance predictive models by incorporating new data and feedback using machine learning techniques.
Keyword: Predictive enrollment forecasting tools