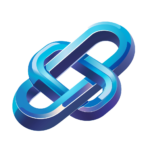
AI Integration for Cost Optimization in Clinical Trials Workflow
AI-driven cost optimization in clinical trials enhances resource allocation minimizes expenses and improves time to market through data analysis and predictive modeling
Category: AI Finance Tools
Industry: Pharmaceuticals
AI-Driven Cost Optimization in Clinical Trials
1. Initial Assessment and Planning
1.1 Define Objectives
Identify the primary goals for cost optimization in clinical trials, such as reducing overall trial expenses, minimizing time to market, and enhancing resource allocation.
1.2 Stakeholder Engagement
Engage relevant stakeholders, including project managers, finance teams, and clinical researchers, to gather insights and establish a collaborative approach.
2. Data Collection and Analysis
2.1 Data Gathering
Collect historical data from previous clinical trials, including budgets, timelines, and resource utilization.
2.2 Data Preprocessing
Utilize AI tools such as Tableau or Microsoft Power BI for data cleaning and transformation to ensure accuracy and consistency.
2.3 Exploratory Data Analysis
Implement AI-driven analytics platforms like IBM Watson Studio to identify patterns and trends in cost drivers across trials.
3. AI Model Development
3.1 Feature Selection
Determine key features that influence costs, such as patient recruitment rates, site performance, and protocol complexity.
3.2 Model Training
Utilize machine learning frameworks such as TensorFlow or PyTorch to develop predictive models that forecast trial costs based on historical data.
3.3 Model Validation
Validate the models using statistical techniques and cross-validation to ensure reliability and accuracy of predictions.
4. Implementation of AI Tools
4.1 Integration with Financial Systems
Integrate AI-driven tools like Oracle’s Siebel Clinical or Medidata Solutions with existing financial systems to automate cost tracking and reporting.
4.2 Real-Time Monitoring
Deploy AI dashboards for real-time monitoring of trial expenses, allowing for immediate adjustments to resource allocation and budgeting.
5. Continuous Improvement
5.1 Feedback Loop
Establish a feedback mechanism to collect insights from stakeholders on the AI tools’ performance and effectiveness in cost optimization.
5.2 Iterative Model Refinement
Continuously refine AI models based on new data and stakeholder feedback to improve accuracy and effectiveness in predicting costs.
5.3 Reporting and Documentation
Generate comprehensive reports using AI tools like QlikView to document cost optimization efforts, outcomes, and lessons learned for future trials.
6. Conclusion
Summarize the outcomes of the AI-driven cost optimization process, highlighting key achievements and areas for further enhancement in clinical trial management.
Keyword: AI cost optimization clinical trials