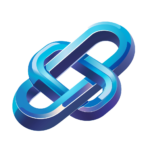
AI Driven Yield Prediction and Crop Planning Workflow Guide
AI-driven yield prediction and crop planning enhance agricultural efficiency by leveraging data collection processing and advanced analytics for optimal results
Category: AI Food Tools
Industry: Agriculture
AI-Based Yield Prediction and Crop Planning
1. Data Collection
1.1 Gather Historical Data
Collect historical data on crop yields, weather patterns, soil conditions, and pest incidents from various sources.
1.2 Sensor and IoT Data
Utilize IoT devices and sensors to gather real-time data on soil moisture, temperature, and other environmental factors.
1.3 Satellite Imagery
Implement satellite imagery to monitor crop health and growth patterns over time.
2. Data Processing
2.1 Data Cleaning
Ensure the collected data is accurate and relevant by removing inconsistencies and errors.
2.2 Data Integration
Integrate data from multiple sources into a centralized database for comprehensive analysis.
3. AI Model Development
3.1 Select AI Algorithms
Choose appropriate machine learning algorithms, such as regression analysis, decision trees, or neural networks, for yield prediction.
3.2 Model Training
Train the AI models using historical data to recognize patterns and make predictions about future yields.
3.3 Model Validation
Validate the model’s accuracy using a separate dataset to ensure reliability in predictions.
4. Yield Prediction
4.1 Predictive Analytics
Utilize AI-driven tools such as IBM Watson or Google AI to analyze data and forecast crop yields based on various scenarios.
4.2 Scenario Analysis
Run simulations to assess the impact of different variables (e.g., weather changes, pest outbreaks) on yield outcomes.
5. Crop Planning
5.1 Crop Selection
Use AI insights to select optimal crops for planting based on predicted yields, market demand, and environmental conditions.
5.2 Resource Allocation
Implement AI tools like AgroStar or Cropio to optimize resource allocation, including water, fertilizers, and labor.
6. Monitoring and Adjustment
6.1 Continuous Monitoring
Leverage AI-powered platforms to continuously monitor crop health and environmental conditions throughout the growing season.
6.2 Adaptive Management
Adjust crop management strategies based on real-time data and AI predictions to maximize yield and minimize risks.
7. Reporting and Analysis
7.1 Performance Reporting
Generate reports using AI analytics tools to evaluate the effectiveness of crop planning and yield outcomes.
7.2 Feedback Loop
Establish a feedback loop to refine AI models and improve future predictions based on new data and outcomes.
Keyword: AI driven crop yield prediction