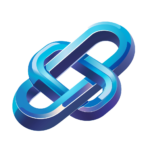
AI Integration in Automated Quality Control and Safety Inspections
AI-driven workflow enhances automated quality control and safety inspections through data collection analysis and compliance monitoring for improved production standards
Category: AI Food Tools
Industry: Food Tech Startups
Automated Quality Control and Safety Inspection
1. Data Collection
1.1 Ingredient Sourcing
Utilize AI-driven tools to gather data on ingredient quality from suppliers. Tools such as IBM Watson can analyze supplier data and predict quality issues based on historical performance.
1.2 Production Monitoring
Implement IoT sensors in production lines to collect real-time data on environmental conditions, such as temperature and humidity. AI platforms like Microsoft Azure IoT can process and analyze this data for anomalies.
2. AI-Driven Quality Analysis
2.1 Visual Inspection
Deploy computer vision systems using AI algorithms to conduct visual inspections of products on the production line. Tools such as Google Cloud Vision can automatically identify defects or contaminants.
2.2 Sensor Data Analysis
Leverage machine learning models to analyze sensor data for deviations from established quality standards. Platforms like TensorFlow can be used to develop predictive models for quality assurance.
3. Safety Inspection Protocols
3.1 Hazard Analysis
Implement AI tools to conduct hazard analysis by evaluating risks based on ingredient data and production processes. Hazard Analysis and Critical Control Points (HACCP) software can be enhanced with AI for better risk assessment.
3.2 Compliance Monitoring
Use AI systems to monitor compliance with safety regulations by cross-referencing production data with legal requirements. Tools like Compliance.ai can automate this process, ensuring adherence to safety standards.
4. Reporting and Documentation
4.1 Automated Reporting
Generate automated reports on quality control and safety inspections using AI tools such as Tableau for data visualization and Google Data Studio for report generation.
4.2 Continuous Improvement Feedback Loop
Establish a feedback loop where AI analyzes inspection results to identify trends and areas for improvement. Utilize Power BI to track performance metrics over time and inform decision-making.
5. Implementation of AI-Driven Solutions
5.1 Training and Development
Conduct training sessions for staff on utilizing AI tools effectively in quality control processes. Incorporate resources from platforms like Coursera to enhance understanding of AI applications.
5.2 Integration with Existing Systems
Ensure seamless integration of AI solutions with existing production management systems. Use APIs from tools like Zapier to connect various software applications for streamlined operations.
6. Review and Optimization
6.1 Performance Evaluation
Regularly evaluate the performance of AI-driven quality control systems to ensure they meet operational goals. Utilize metrics such as defect rates and compliance scores for assessment.
6.2 Continuous AI Model Refinement
Continuously refine AI models based on new data and insights gained from inspections. Implement machine learning techniques to adapt to evolving quality standards and safety regulations.
Keyword: AI driven quality control systems