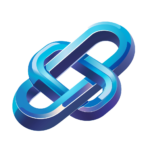
AI Driven Drug Discovery Pipeline Enhancing Efficiency and Innovation
AI-driven drug discovery pipeline enhances efficiency and reduces costs by utilizing advanced tools for target identification compound screening and clinical trial design
Category: AI Health Tools
Industry: Biotechnology firms
AI-Driven Drug Discovery Pipeline
1. Target Identification
Utilize AI algorithms to analyze biological data and identify potential drug targets.
- Tools: DeepMind’s AlphaFold for protein structure prediction.
- Example: Integrating genomic data with machine learning models to pinpoint disease-related genes.
2. Compound Screening
Employ AI to predict the efficacy of various compounds against identified targets.
- Tools: Atomwise for virtual screening using deep learning.
- Example: Using convolutional neural networks (CNNs) to evaluate compound libraries.
3. Lead Optimization
Refine lead compounds through AI-driven simulations and predictive modeling.
- Tools: ChemAxon for cheminformatics and molecular modeling.
- Example: Implementing reinforcement learning to optimize molecular properties.
4. Preclinical Testing
Utilize AI to analyze preclinical data and predict clinical outcomes.
- Tools: IBM Watson for Drug Discovery for data integration and analysis.
- Example: Machine learning models predicting toxicity and pharmacokinetics.
5. Clinical Trial Design
Leverage AI to design and optimize clinical trials for efficiency and effectiveness.
- Tools: Medidata Solutions for trial design and patient recruitment analytics.
- Example: Using predictive analytics to identify suitable patient populations.
6. Data Analysis and Interpretation
Implement AI to analyze clinical trial data and derive actionable insights.
- Tools: BioSymetrics for advanced analytics and data visualization.
- Example: Utilizing natural language processing (NLP) to interpret clinical notes and reports.
7. Regulatory Submission
Streamline the regulatory submission process with AI-supported documentation and compliance checks.
- Tools: Veeva Vault for regulatory document management.
- Example: Automating data extraction from clinical trial reports for submission packages.
8. Post-Market Surveillance
Employ AI to monitor drug performance and safety in the market.
- Tools: Oracle’s Argus for pharmacovigilance and adverse event reporting.
- Example: Machine learning models analyzing real-world data for safety signals.
Conclusion
The integration of AI in the drug discovery pipeline enhances efficiency, reduces costs, and accelerates the development of innovative therapies. Biotechnology firms can leverage these advanced tools to stay competitive in the evolving landscape of pharmaceutical development.
Keyword: AI driven drug discovery process