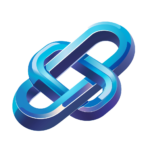
AI Driven Predictive Maintenance Workflow for Biotech Equipment
Discover how AI-driven predictive maintenance enhances biotech manufacturing equipment efficiency through real-time data collection analysis and proactive scheduling
Category: AI Health Tools
Industry: Biotechnology firms
Predictive Maintenance for Biotech Manufacturing Equipment
1. Data Collection
1.1 Identify Equipment
List all biotech manufacturing equipment that requires predictive maintenance.
1.2 Sensor Installation
Install IoT sensors on equipment to collect real-time data on performance metrics, such as temperature, vibration, and pressure.
1.3 Data Integration
Integrate data from sensors with existing systems (e.g., ERP, MES) to create a centralized data repository.
2. Data Analysis
2.1 Data Preprocessing
Clean and preprocess the collected data to remove noise and ensure quality.
2.2 AI Model Selection
Select appropriate AI models for predictive analytics, such as:
- Regression Analysis
- Time-Series Forecasting
- Machine Learning Algorithms (e.g., Random Forest, Neural Networks)
2.3 Tool Implementation
Utilize AI-driven products such as:
- IBM Watson IoT: For real-time data analysis and predictive insights.
- Siemens MindSphere: To connect equipment and analyze operational data.
- Uptake: For AI-driven maintenance insights and recommendations.
3. Predictive Modeling
3.1 Model Training
Train selected AI models using historical data to identify patterns and predict potential equipment failures.
3.2 Model Validation
Validate the accuracy of the models through back-testing with historical incidents.
4. Maintenance Planning
4.1 Failure Prediction
Utilize AI-generated insights to predict equipment failures before they occur.
4.2 Scheduling Maintenance
Develop a maintenance schedule based on predictive insights, prioritizing high-risk equipment.
4.3 Resource Allocation
Allocate necessary resources (e.g., parts, personnel) based on the predictive maintenance schedule.
5. Implementation and Monitoring
5.1 Execute Maintenance Tasks
Conduct maintenance activities as per the schedule, ensuring minimal disruption to production.
5.2 Continuous Monitoring
Monitor equipment performance post-maintenance to ensure effectiveness and capture new data for future analysis.
6. Feedback Loop
6.1 Performance Review
Review the performance of the AI models and maintenance outcomes regularly.
6.2 Model Refinement
Refine AI models based on new data and insights to improve predictive accuracy.
6.3 Stakeholder Engagement
Engage stakeholders with regular reports on maintenance effectiveness and predictive insights to drive continuous improvement.
Keyword: predictive maintenance biotech equipment