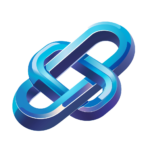
AI Driven Customer Dispute Resolution and Risk Assessment Workflow
AI-driven customer dispute resolution streamlines inquiries categorizes disputes assesses legal risks and enhances communication for effective resolutions.
Category: AI Legal Tools
Industry: Retail and E-commerce
AI-Driven Customer Dispute Resolution and Legal Risk Assessment
1. Initial Customer Inquiry
1.1. Customer Submission
Customers submit their disputes through an online portal or customer service hotline.
1.2. AI Chatbot Engagement
Utilize AI-driven chatbots (e.g., Zendesk, Ada) to interact with customers, gather initial details, and categorize the dispute type.
2. Dispute Categorization and Analysis
2.1. Data Extraction
Employ Natural Language Processing (NLP) tools (e.g., IBM Watson, Google Cloud Natural Language) to extract key information from customer submissions.
2.2. Dispute Classification
AI algorithms classify disputes into categories (e.g., product defect, shipping issue, refund request) for streamlined processing.
3. Resolution Pathway Determination
3.1. Risk Assessment
Utilize AI models (e.g., LexPredict, Legal Robot) to assess the legal risks associated with each dispute category.
3.2. Suggested Resolutions
AI systems generate suggested resolutions based on historical data and successful outcomes from similar cases.
4. Customer Communication
4.1. Automated Response Generation
Implement AI tools (e.g., ChatGPT, Salesforce Einstein) to draft personalized responses to customers, outlining potential resolutions and next steps.
4.2. Follow-Up Engagement
Schedule follow-up communications through automated systems to ensure customer satisfaction and resolution confirmation.
5. Dispute Resolution Execution
5.1. Resolution Implementation
Execute the resolution as determined by the AI system, whether it involves refunds, replacements, or other remedies.
5.2. Documentation and Record Keeping
Utilize document automation tools (e.g., HotDocs, Contract Express) to create and store records of the dispute and resolution process.
6. Post-Resolution Analysis
6.1. Data Collection
Aggregate data on resolved disputes for further analysis and reporting.
6.2. Performance Metrics Evaluation
Leverage AI analytics tools (e.g., Tableau, Power BI) to evaluate the effectiveness of the dispute resolution process and identify areas for improvement.
7. Continuous Improvement
7.1. Feedback Loop Integration
Integrate customer feedback into the AI system to refine algorithms and improve future dispute resolution processes.
7.2. Training and Model Updates
Regularly update AI models with new data to enhance accuracy in risk assessments and resolution strategies.
Keyword: AI customer dispute resolution system