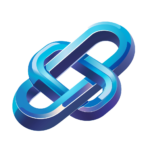
AI Integration for Enhanced Quality Control in Manufacturing
AI-assisted quality control enhances manufacturing processes by integrating real-time data analysis and machine learning for improved product quality and efficiency.
Category: AI Media Tools
Industry: Automotive
AI-Assisted Quality Control in Manufacturing Processes
1. Initial Assessment
1.1 Define Quality Standards
Establish the quality benchmarks that products must meet, including specifications for materials, dimensions, and performance.
1.2 Identify Key Processes
Determine the critical manufacturing processes where quality control will be implemented, such as assembly, painting, and inspection.
2. Data Collection
2.1 Sensor Integration
Utilize IoT sensors to gather real-time data from manufacturing equipment and processes. Examples include:
- Temperature and humidity sensors
- Vibration sensors on machinery
2.2 Historical Data Analysis
Compile historical production data to identify trends and common defects. This can be achieved using tools like:
- Tableau for data visualization
- Microsoft Power BI for business analytics
3. AI Implementation
3.1 Machine Learning Models
Develop machine learning algorithms to analyze collected data and predict potential quality issues. Tools such as:
- TensorFlow for building models
- RapidMiner for data preparation and model deployment
can be utilized to enhance predictive capabilities.
3.2 Computer Vision Systems
Implement AI-driven computer vision systems to inspect products for defects in real-time. Examples include:
- Cognex Vision Systems for automated inspection
- Google Cloud Vision for image analysis
4. Quality Control Execution
4.1 Automated Inspection
Deploy AI tools for automated inspections, reducing human error and increasing efficiency. This includes:
- Using drones equipped with cameras for large-scale inspections
- Robotic arms with vision capabilities for precise assembly checks
4.2 Feedback Loop
Establish a feedback mechanism to continuously improve the quality control process. Integrate AI tools that allow for:
- Real-time reporting dashboards
- Automated alerts for deviations from quality standards
5. Continuous Improvement
5.1 Data-Driven Insights
Leverage AI analytics tools to derive insights from quality control data. Tools such as:
- IBM Watson for advanced analytics
- QlikView for interactive visual analytics
can help in identifying root causes of defects.
5.2 Training and Development
Implement training programs for staff based on insights gained from AI analysis. Focus on:
- Best practices for quality assurance
- Utilization of AI tools in daily operations
6. Review and Optimization
6.1 Performance Metrics
Regularly review quality control performance metrics to assess the effectiveness of AI tools and processes.
6.2 Process Refinement
Continuously refine manufacturing processes based on AI-driven insights to enhance product quality and operational efficiency.
Keyword: AI quality control in manufacturing