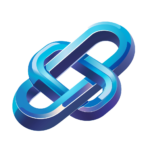
AI Integration in Clinical Decision Support Workflow for Healthcare
AI-powered clinical decision support systems enhance healthcare by improving diagnostic accuracy treatment planning and patient management through advanced AI tools
Category: AI Media Tools
Industry: Healthcare
AI-Powered Clinical Decision Support System
1. Workflow Overview
This workflow outlines the integration of AI-powered tools into clinical decision-making processes within healthcare settings. The aim is to enhance diagnostic accuracy, treatment planning, and patient management through the utilization of advanced AI technologies.
2. Key Components
2.1 Data Collection
Gather patient data from various sources including electronic health records (EHR), lab results, and imaging studies.
2.2 Data Preprocessing
Utilize AI tools to clean and standardize data to ensure accuracy and consistency. Tools such as DataRobot and Trifacta can be employed for this purpose.
2.3 AI Model Development
Develop predictive models using machine learning algorithms. Tools like TensorFlow and PyTorch can be utilized to create models that predict patient outcomes based on historical data.
2.4 Integration with Clinical Workflows
Integrate AI models into existing clinical workflows through APIs. This allows seamless access to AI-driven insights during patient consultations.
3. Implementation of AI Tools
3.1 Clinical Decision Support Systems (CDSS)
Implement AI-powered CDSS such as IBM Watson Health or Epic’s AI tools to provide real-time recommendations to healthcare providers.
3.2 Imaging Analysis
Utilize AI-driven imaging tools like Aidoc or Zebra Medical Vision for automated analysis of radiological images, assisting in quicker and more accurate diagnoses.
3.3 Natural Language Processing (NLP)
Employ NLP tools such as Google Cloud Natural Language to analyze unstructured clinical notes, extracting valuable insights that inform treatment plans.
4. Continuous Monitoring and Evaluation
4.1 Performance Tracking
Regularly monitor the performance of AI models using metrics such as accuracy, sensitivity, and specificity to ensure ongoing effectiveness.
4.2 User Feedback
Collect feedback from healthcare providers on the usability and impact of AI tools to drive continuous improvement.
5. Training and Support
5.1 Staff Training
Provide comprehensive training for healthcare staff on the use of AI tools and interpretation of AI-generated insights.
5.2 Technical Support
Establish a dedicated support team to assist with technical issues and ensure smooth operation of AI systems within the clinical environment.
6. Compliance and Ethics
6.1 Regulatory Compliance
Ensure all AI implementations comply with healthcare regulations such as HIPAA and GDPR to protect patient data.
6.2 Ethical Considerations
Address ethical implications of AI in healthcare, ensuring transparency and fairness in AI-driven decisions.
7. Conclusion
The integration of AI-powered clinical decision support systems represents a transformative opportunity for healthcare providers to enhance patient care. By leveraging advanced AI tools, healthcare organizations can improve diagnostic accuracy, streamline workflows, and ultimately deliver better patient outcomes.
Keyword: AI clinical decision support system