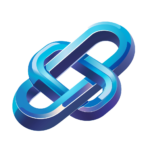
AI Integration in Predictive Maintenance for Pharma Manufacturing
AI-driven predictive maintenance enhances pharmaceutical manufacturing by ensuring equipment reliability compliance and operational efficiency through data analysis and automation
Category: AI Networking Tools
Industry: Pharmaceuticals and Biotechnology
Predictive Maintenance for Pharmaceutical Manufacturing
1. Introduction to Predictive Maintenance
Predictive maintenance utilizes data analysis tools and techniques to detect anomalies in equipment operation and potential defects in manufacturing processes. This workflow outlines the integration of AI networking tools to enhance predictive maintenance in pharmaceutical manufacturing.
2. Data Collection
2.1 Equipment Sensors
Install IoT sensors on critical manufacturing equipment to continuously monitor performance metrics such as temperature, vibration, and pressure.
2.2 Data Integration
Utilize AI-driven platforms like IBM Watson IoT or Siemens MindSphere to aggregate data from various sources including ERP systems, SCADA systems, and manufacturing execution systems (MES).
3. Data Analysis
3.1 Anomaly Detection
Implement machine learning algorithms to analyze historical and real-time data for identifying patterns and anomalies. Tools such as DataRobot or Google AI Platform can be employed for this purpose.
3.2 Predictive Modeling
Develop predictive models using AI frameworks like TensorFlow or Pytorch to forecast equipment failures and maintenance needs based on collected data.
4. Maintenance Scheduling
4.1 Automated Alerts
Set up automated alerts through AI tools like Microsoft Azure Machine Learning to notify maintenance teams of potential issues before they escalate.
4.2 Resource Allocation
Utilize AI-driven optimization tools to allocate resources effectively for maintenance tasks, ensuring minimal disruption to manufacturing operations.
5. Implementation of Maintenance Actions
5.1 Predictive Maintenance Execution
Carry out maintenance activities based on AI-generated insights, ensuring that actions are data-driven and timely.
5.2 Documentation and Compliance
Utilize electronic lab notebooks (ELNs) and document management systems to maintain records of maintenance actions taken, ensuring compliance with regulatory standards.
6. Continuous Improvement
6.1 Performance Monitoring
Continuously monitor equipment performance post-maintenance using AI tools to evaluate the effectiveness of maintenance strategies.
6.2 Feedback Loop
Integrate feedback mechanisms to refine predictive models and maintenance schedules based on performance data and outcomes.
7. Conclusion
The implementation of AI networking tools in predictive maintenance for pharmaceutical manufacturing not only enhances operational efficiency but also ensures compliance and quality assurance throughout the production process.
Keyword: Predictive maintenance in pharmaceuticals