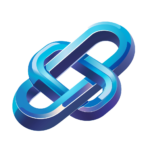
AI Integration in Real-Time Battle Damage Assessment Workflow
Real-Time Battle Damage Assessment Network leverages AI tools for efficient damage assessment in aerospace and defense enhancing accuracy and operational effectiveness
Category: AI Networking Tools
Industry: Aerospace and Defense
Real-Time Battle Damage Assessment Network
1. Objective
The primary objective of the Real-Time Battle Damage Assessment Network is to utilize AI networking tools to enhance the efficiency and accuracy of damage assessment in aerospace and defense operations.
2. Workflow Overview
This workflow outlines the steps involved in conducting real-time battle damage assessments using AI-driven tools and technologies.
2.1 Key Components
- Data Collection
- Data Analysis
- Damage Assessment Reporting
- Feedback Loop for Continuous Improvement
3. Workflow Steps
3.1 Data Collection
Utilize various data sources to gather information related to battle damage.
- AI-Driven Surveillance Drones: Deploy drones equipped with imaging technology to capture real-time data.
- Satellite Imagery: Use high-resolution satellite images to assess the impact of engagements.
- Sensor Networks: Implement ground-based sensors to monitor damage across various operational theaters.
3.2 Data Analysis
Leverage AI algorithms to process and analyze the collected data.
- Machine Learning Models: Apply machine learning techniques to identify patterns and assess damage severity.
- Image Recognition Software: Use AI-driven tools like Google Cloud Vision or AWS Rekognition to automate the identification of damage in images.
- Predictive Analytics: Implement AI models to predict potential future damage based on current assessments.
3.3 Damage Assessment Reporting
Generate comprehensive reports based on the analyzed data.
- Automated Reporting Tools: Utilize platforms such as Tableau or Power BI to create visual dashboards that present damage assessments in real-time.
- AI-Enhanced Documentation: Use natural language processing (NLP) tools to generate detailed reports from data insights.
3.4 Feedback Loop for Continuous Improvement
Incorporate feedback mechanisms to refine the assessment process.
- User Feedback: Collect input from field operatives to improve AI algorithms and data collection methods.
- Performance Metrics: Analyze the effectiveness of damage assessments and adjust AI tools accordingly.
- Ongoing Training: Continuously train AI models with new data to enhance accuracy and efficiency.
4. Conclusion
The Real-Time Battle Damage Assessment Network integrates advanced AI networking tools to streamline the damage assessment process in aerospace and defense. By utilizing state-of-the-art technologies, organizations can achieve more accurate assessments and improve operational effectiveness.
Keyword: real-time battle damage assessment