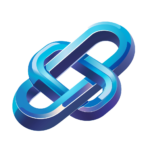
Automated Pest and Disease Detection with AI Integration
Automated pest and disease detection workflow enhances crop management through AI-driven image analysis real-time monitoring and actionable insights for farmers
Category: AI News Tools
Industry: Agriculture
Automated Pest and Disease Detection Workflow
1. Data Collection
1.1. Image Acquisition
Utilize drones equipped with high-resolution cameras to capture images of crops from various angles. This allows for comprehensive coverage and real-time monitoring.
1.2. Sensor Deployment
Install IoT sensors in the fields to collect environmental data such as humidity, temperature, and soil conditions, which can influence pest and disease occurrence.
2. Data Processing
2.1. Image Preprocessing
Apply image enhancement techniques to improve the quality of the images captured. This may include noise reduction and contrast adjustment.
2.2. Data Integration
Integrate data from multiple sources (drones, sensors, and historical data) into a centralized database for comprehensive analysis.
3. AI Model Development
3.1. Training AI Algorithms
Utilize machine learning frameworks such as TensorFlow or PyTorch to train models on labeled datasets of pest and disease images.
Example Tools: PlantVillage Nuru – an AI tool that identifies plant diseases using image recognition.
3.2. Model Validation
Validate the AI models using a separate dataset to ensure accuracy and reliability in real-world applications.
4. Detection and Analysis
4.1. Real-Time Monitoring
Implement AI-driven image recognition tools to analyze incoming data in real-time. This allows for immediate detection of pests and diseases.
Example Tools: AgriBot – an AI-powered robot that can identify and treat plant diseases on-site.
4.2. Risk Assessment
Utilize predictive analytics to assess the risk of pest and disease outbreaks based on environmental conditions and historical data.
5. Reporting and Action
5.1. Automated Reporting
Generate automated reports that summarize findings, including detected pests or diseases, affected areas, and recommended actions.
5.2. Actionable Insights
Provide farmers with actionable insights through mobile applications or dashboards, enabling them to make informed decisions regarding pest management strategies.
Example Tools: CropX – a platform that offers data-driven insights for irrigation and pest management.
6. Continuous Improvement
6.1. Feedback Loop
Establish a feedback mechanism where farmers can report the effectiveness of the suggested actions, allowing for continuous model improvement.
6.2. Model Retraining
Regularly update and retrain AI models with new data to enhance detection capabilities and adapt to changing agricultural conditions.
Keyword: automated pest detection workflow