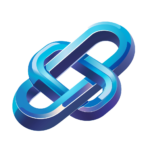
AI Driven Churn Prediction and Retention Workflow Explained
AI-driven churn prediction enhances customer retention through data collection analysis and personalized strategies to reduce churn rates and improve satisfaction
Category: AI Relationship Tools
Industry: Telecommunications
AI-Driven Churn Prediction and Retention
1. Data Collection
1.1 Customer Data
Gather comprehensive customer data including demographics, usage patterns, billing history, and customer service interactions.
1.2 External Data
Integrate external data sources such as market trends, competitor analysis, and social media sentiment.
2. Data Preprocessing
2.1 Data Cleaning
Utilize tools like OpenRefine to clean and standardize data.
2.2 Feature Engineering
Identify relevant features that may indicate churn, such as service usage frequency and complaint history.
3. AI Model Development
3.1 Model Selection
Choose appropriate machine learning models such as Random Forest, Gradient Boosting, or Neural Networks for churn prediction.
3.2 Training the Model
Use platforms like TensorFlow or Scikit-learn to train the selected models on historical data.
4. Churn Prediction
4.1 Implementation of Predictive Analytics
Deploy the trained model using tools such as IBM Watson or Azure Machine Learning to generate churn predictions.
4.2 Real-time Monitoring
Implement real-time monitoring systems to continuously assess customer behavior and predict churn likelihood.
5. Customer Segmentation
5.1 Identifying At-Risk Customers
Segment customers based on churn risk scores to tailor retention strategies.
5.2 Behavioral Analysis
Utilize AI tools such as Tableau or Power BI for visualizing customer segments and behaviors.
6. Retention Strategy Development
6.1 Personalized Communication
Leverage AI-driven CRM tools like Salesforce Einstein to create personalized marketing campaigns aimed at at-risk customers.
6.2 Incentives and Offers
Design targeted incentives, such as discounts or loyalty programs, based on customer profiles and preferences.
7. Implementation of Retention Strategies
7.1 Multi-Channel Engagement
Engage customers through various channels including email, SMS, and social media using tools like HubSpot.
7.2 Feedback Mechanism
Establish feedback loops to gather customer responses and adjust strategies accordingly.
8. Performance Monitoring and Adjustment
8.1 KPI Tracking
Monitor key performance indicators (KPIs) such as churn rate, customer satisfaction score, and retention rate using analytics platforms.
8.2 Continuous Improvement
Utilize insights gained from data analysis to continuously refine churn prediction models and retention strategies.
9. Reporting and Analysis
9.1 Regular Reporting
Generate regular reports to assess the effectiveness of churn prediction and retention efforts.
9.2 Stakeholder Presentation
Present findings and strategic recommendations to stakeholders to ensure alignment and support for ongoing initiatives.
Keyword: AI-driven churn prediction strategies