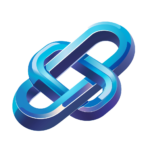
AI Driven Fraud Detection and Revenue Protection Workflow
AI-driven workflow enhances fraud detection and revenue protection through data collection preprocessing model development real-time monitoring and compliance adherence
Category: AI Relationship Tools
Industry: Energy and Utilities
Fraud Detection and Revenue Protection
1. Data Collection
1.1 Customer Data
Gather comprehensive customer data including demographics, account history, and payment behaviors.
1.2 Consumption Data
Collect energy consumption data through smart meters and IoT devices to analyze usage patterns.
2. Data Preprocessing
2.1 Data Cleaning
Utilize AI algorithms to identify and rectify inconsistencies or inaccuracies in the collected data.
2.2 Data Integration
Integrate data from various sources such as CRM systems, billing software, and external databases.
3. Fraud Detection Model Development
3.1 AI Model Selection
Select appropriate AI models such as supervised learning algorithms (e.g., Random Forest, Support Vector Machines) or unsupervised learning techniques (e.g., clustering algorithms).
3.2 Tool Implementation
Implement AI-driven tools such as:
- IBM Watson: For predictive analytics and anomaly detection.
- Google Cloud AutoML: To build custom models tailored to specific fraud detection needs.
- Microsoft Azure Machine Learning: For scalable model deployment and monitoring.
4. Real-Time Monitoring
4.1 Anomaly Detection
Utilize AI algorithms to continuously monitor real-time data for unusual patterns that may indicate fraud.
4.2 Alert System
Establish an automated alert system that notifies relevant personnel of potential fraudulent activities.
5. Investigation and Response
5.1 Case Management
Utilize AI-driven case management tools to streamline the investigation process of flagged accounts.
5.2 Decision Support
Employ AI systems to provide decision support, recommending actions based on historical data and outcomes.
6. Reporting and Analytics
6.1 Performance Metrics
Generate reports on fraud detection performance, including metrics such as detection rates, false positives, and recovery rates.
6.2 Continuous Improvement
Utilize insights from reports to refine AI models and improve detection accuracy over time.
7. Customer Engagement
7.1 Communication Strategies
Implement AI-driven communication tools to engage with customers regarding their accounts and potential fraud alerts.
7.2 Feedback Loop
Establish a feedback mechanism to gather customer input on the effectiveness of fraud detection measures.
8. Compliance and Regulatory Adherence
8.1 Policy Alignment
Ensure that all fraud detection processes align with industry regulations and compliance standards.
8.2 Auditing
Conduct regular audits using AI tools to ensure adherence to compliance requirements and identify areas for improvement.
Keyword: AI fraud detection workflow