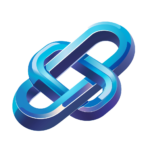
AI-Driven Workflow for Enhanced Drug Safety Signal Detection
AI-driven drug safety signal detection enhances data collection preprocessing and validation while automating risk assessment and reporting for improved patient safety
Category: AI Relationship Tools
Industry: Pharmaceuticals and Biotechnology
AI-Enhanced Drug Safety Signal Detection
1. Data Collection
1.1 Identify Data Sources
Gather data from various sources including clinical trials, electronic health records (EHR), social media, and adverse event reporting systems.
1.2 Utilize AI Tools for Data Aggregation
Implement AI-driven platforms such as IBM Watson for Drug Discovery and Oracle’s Argus Safety to aggregate and normalize data from disparate sources.
2. Data Preprocessing
2.1 Data Cleaning
Employ machine learning algorithms to identify and rectify inconsistencies or errors in the data.
2.2 Data Transformation
Utilize Natural Language Processing (NLP) tools, such as Google’s BERT, to convert unstructured data into structured formats suitable for analysis.
3. Signal Detection
3.1 Develop AI Models
Create predictive models using supervised learning techniques to identify potential safety signals from the processed data.
3.2 Implement AI Tools for Signal Detection
Use AI-driven products like SAS Drug Development and Bioclinica’s Clinical Data Management System to automate the detection of safety signals.
4. Signal Validation
4.1 Manual Review
Conduct a manual review of identified signals using expert panels to validate AI-generated findings.
4.2 Utilize AI for Validation
Incorporate AI tools such as Aetion and Flatiron Health to cross-validate signals against real-world evidence and historical data.
5. Risk Assessment
5.1 Risk Characterization
Analyze the validated signals to characterize the risk associated with the drug using AI-based risk assessment models.
5.2 Decision Support Systems
Leverage AI-driven decision support tools like IBM Watson Health to assist in evaluating the clinical significance of identified risks.
6. Reporting and Communication
6.1 Generate Reports
Automate the generation of safety reports using AI tools to ensure compliance with regulatory requirements.
6.2 Stakeholder Communication
Utilize AI platforms for effective communication of findings to stakeholders, including healthcare providers and regulatory bodies.
7. Continuous Monitoring
7.1 Implement Real-Time Monitoring Systems
Deploy real-time monitoring systems powered by AI to continuously assess drug safety signals post-market.
7.2 Feedback Loop
Create a feedback mechanism using AI analytics to refine and improve the signal detection process over time.
Keyword: AI-driven drug safety detection