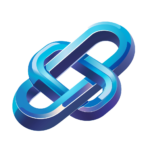
AI Integrated Network Troubleshooting Workflow for Efficient Solutions
Discover an AI-driven network troubleshooting assistant that enhances problem identification diagnosis resolution and continuous improvement for optimal performance
Category: AI Relationship Tools
Industry: Telecommunications
Intelligent Network Troubleshooting Assistant
1. Problem Identification
1.1 Data Collection
Utilize AI-driven analytics tools such as Splunk or IBM Watson to gather real-time data from network devices, user reports, and performance metrics.
1.2 Anomaly Detection
Implement machine learning algorithms to analyze historical data and identify anomalies in network performance. Tools like Darktrace can be employed for real-time threat detection.
2. Diagnosis Phase
2.1 Automated Diagnostics
Leverage AI-based diagnostic tools such as NetBrain to automatically assess the network topology and pinpoint potential failure points.
2.2 Root Cause Analysis
Use AI algorithms to correlate data from various sources, enabling tools like Moogsoft to provide insights into the root causes of issues.
3. Resolution Planning
3.1 Suggested Solutions
AI systems can recommend solutions based on past incidents and resolutions. Tools like ServiceNow can facilitate this process by providing a knowledge base of previous troubleshooting steps.
3.2 Impact Assessment
Utilize predictive analytics to evaluate the potential impact of proposed solutions on network performance. AI tools such as Microsoft Azure Machine Learning can assist in modeling these scenarios.
4. Implementation
4.1 Automated Remediation
Employ AI-driven automation tools such as Cisco DNA Center to implement fixes without human intervention, reducing downtime and improving efficiency.
4.2 Monitoring Post-Implementation
After implementing solutions, utilize continuous monitoring tools like New Relic to ensure that the network is stable and performing as expected.
5. Feedback Loop
5.1 Performance Review
Conduct a performance review using AI analytics to assess the effectiveness of the implemented solutions, leveraging tools like Tableau for visualization.
5.2 Knowledge Base Update
Update the organization’s knowledge base with insights gained from the troubleshooting process, ensuring that AI tools like Zendesk are utilized for documentation and future reference.
6. Continuous Improvement
6.1 AI Model Training
Regularly retrain AI models with new data to improve accuracy and efficiency in future troubleshooting. Tools like TensorFlow can be used for this purpose.
6.2 Stakeholder Feedback
Gather feedback from network operators and stakeholders to refine the troubleshooting process, ensuring that AI tools are aligned with user needs and expectations.
Keyword: AI network troubleshooting assistant