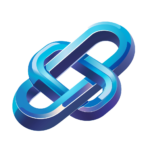
AI Powered Predictive Maintenance Scheduling Workflow Guide
AI-driven predictive maintenance scheduling enhances vehicle performance by integrating data analyzing potential failures and automating maintenance plans for efficiency
Category: AI Relationship Tools
Industry: Automotive
Predictive Maintenance Scheduling with AI
1. Data Collection
1.1 Identify Data Sources
Collect data from various sources including:
- Vehicle sensors
- Telematics systems
- Maintenance logs
1.2 Data Integration
Utilize AI-driven tools such as:
- IBM Watson IoT: Integrates data from multiple sources for comprehensive analysis.
- Microsoft Azure IoT: Collects and processes data in real-time.
2. Data Analysis
2.1 Predictive Analytics
Implement machine learning algorithms to analyze historical data and predict potential failures.
2.2 AI Tools for Analysis
Utilize the following AI-driven products:
- TensorFlow: For building predictive models.
- RapidMiner: For data mining and predictive analytics.
3. Maintenance Scheduling
3.1 Automated Scheduling
Create a maintenance schedule based on predictive analysis outcomes using AI tools.
3.2 Tools for Scheduling
Examples of AI-driven scheduling tools include:
- UpKeep: Provides automated maintenance scheduling.
- Fiix: Uses AI to optimize maintenance workflows.
4. Implementation and Monitoring
4.1 Execute Maintenance Plans
Deploy maintenance plans based on the AI-generated schedule.
4.2 Continuous Monitoring
Utilize AI tools for ongoing monitoring of vehicle performance:
- Geotab: Monitors vehicle health and performance metrics.
- Fleet Complete: Provides real-time tracking and alerts for maintenance needs.
5. Feedback Loop
5.1 Data Feedback
Collect data post-maintenance to refine predictive models and improve accuracy.
5.2 Continuous Improvement
Utilize AI to analyze feedback and enhance future predictive maintenance strategies.
Keyword: AI predictive maintenance scheduling