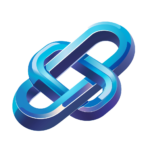
AI Driven Demand Response and Load Balancing Workflow Guide
AI-driven demand response and load balancing workflow enhances energy efficiency through data analysis forecasting and consumer engagement strategies
Category: AI Research Tools
Industry: Energy and Utilities
Demand Response and Load Balancing Workflow
1. Identify Demand Response Opportunities
1.1 Data Collection
Utilize AI-powered data analytics tools to gather historical energy consumption data from various sources, including smart meters and IoT devices.
1.2 Analyze Consumption Patterns
Implement machine learning algorithms to identify peak demand periods and consumer behavior trends. Tools such as IBM Watson IoT and Google Cloud AI can be leveraged for this analysis.
2. Develop Load Balancing Strategies
2.1 Forecasting Demand
Employ AI-driven forecasting tools like EnergyHub and AutoGrid to predict future energy demand based on historical data and external factors such as weather conditions.
2.2 Create Load Balancing Models
Utilize optimization algorithms to develop models that balance load distribution across the grid. Tools such as MATLAB and Plexos can assist in creating these models.
3. Implement Demand Response Programs
3.1 Program Design
Design demand response programs that incentivize consumers to reduce or shift their energy usage during peak periods. AI tools can help in tailoring these programs based on consumer profiles.
3.2 Consumer Engagement
Utilize AI chatbots and mobile applications to communicate with consumers regarding demand response initiatives. Tools like Drift and Zendesk can facilitate effective engagement.
4. Monitor and Optimize Performance
4.1 Real-Time Monitoring
Implement AI-powered monitoring systems to provide real-time insights into energy consumption and demand response program performance. Tools such as Schneider Electric’s EcoStruxure can be utilized for this purpose.
4.2 Continuous Improvement
Use AI analytics to assess the effectiveness of demand response strategies and make data-driven adjustments. Platforms like Tableau and Power BI can be employed for visualization and reporting.
5. Evaluate and Report Outcomes
5.1 Performance Metrics
Establish key performance indicators (KPIs) to measure the success of demand response and load balancing initiatives, utilizing AI tools for accurate data analysis.
5.2 Reporting
Generate comprehensive reports on program outcomes and insights for stakeholders using AI-driven reporting tools like Qlik Sense.
Keyword: AI demand response strategies