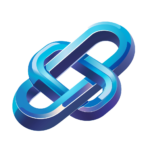
AI Driven Predictive Maintenance for Critical Infrastructure Security
AI-driven predictive maintenance enhances security for critical infrastructure by utilizing real-time data analysis to prevent downtime and ensure compliance
Category: AI Security Tools
Industry: Energy and Utilities
Predictive Maintenance for Critical Infrastructure Security
1. Define Objectives
1.1 Identify Critical Infrastructure
Assess and categorize the critical infrastructure components within energy and utilities, such as power plants, transmission lines, and water treatment facilities.
1.2 Establish Security Goals
Determine the security goals aimed at protecting these infrastructures, including minimizing downtime, preventing unauthorized access, and ensuring compliance with regulations.
2. Data Collection and Integration
2.1 Sensor Deployment
Install IoT sensors on critical assets to collect real-time data on operational parameters, environmental conditions, and potential security threats.
2.2 Data Aggregation
Utilize platforms like AWS IoT or Microsoft Azure to aggregate data from various sources, ensuring seamless integration for analysis.
3. AI Model Development
3.1 Data Preprocessing
Clean and preprocess the collected data to enhance the quality and relevance for AI model training.
3.2 Model Selection
Choose appropriate machine learning algorithms, such as supervised learning for anomaly detection or reinforcement learning for predictive analysis.
3.3 Tool Utilization
Implement AI-driven tools such as IBM Watson for predictive analytics or Google Cloud AI for machine learning model development.
4. Predictive Analysis
4.1 Anomaly Detection
Utilize AI models to identify deviations from normal operational patterns that may indicate potential security threats.
4.2 Predictive Maintenance Scheduling
Leverage AI insights to schedule maintenance activities proactively, reducing the likelihood of equipment failure and enhancing security measures.
5. Implementation of Security Protocols
5.1 Automated Response Systems
Incorporate AI-driven automation tools, such as Splunk or Darktrace, to respond to detected anomalies in real-time.
5.2 Security Policy Updates
Regularly update security protocols based on insights gained from predictive analysis to address new vulnerabilities.
6. Continuous Monitoring and Improvement
6.1 Performance Evaluation
Continuously assess the effectiveness of predictive maintenance and security measures through KPIs and performance metrics.
6.2 Iterative Model Refinement
Refine AI models based on ongoing data collection and feedback to improve accuracy and reliability in predictive maintenance.
7. Reporting and Compliance
7.1 Generate Reports
Create comprehensive reports detailing maintenance activities, security incidents, and compliance with regulatory standards.
7.2 Stakeholder Communication
Communicate findings and recommendations to stakeholders to ensure transparency and support for ongoing initiatives.
Keyword: Predictive maintenance for infrastructure security