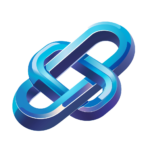
AI Driven Threat Intelligence Workflow for Enhanced Cybersecurity
AI-driven threat intelligence enhances cybersecurity by automating data collection analysis and response improving detection speed and accuracy
Category: AI Security Tools
Industry: Cybersecurity
AI-Driven Threat Intelligence Gathering and Analysis
1. Define Objectives
1.1 Identify Key Threats
Assess the organization’s specific cybersecurity threats, such as phishing, malware, or insider threats.
1.2 Establish Goals
Set clear objectives for threat intelligence gathering, including detection speed, accuracy, and response time.
2. Data Collection
2.1 Utilize AI Tools for Data Aggregation
Implement AI-driven tools such as Recorded Future and ThreatConnect to aggregate data from various sources, including dark web forums, social media, and threat databases.
2.2 Automate Data Ingestion
Employ Splunk or IBM QRadar to automate the ingestion of logs and events from security devices and endpoints.
3. Data Processing
3.1 Normalize and Enrich Data
Use AI algorithms to normalize data formats and enrich datasets with contextual information, leveraging tools such as Maltego.
3.2 Apply Machine Learning Models
Implement machine learning models to classify and prioritize threats based on historical data and patterns.
4. Threat Analysis
4.1 Conduct Behavioral Analysis
Utilize AI systems like Cylance to perform behavioral analysis of network traffic and user activities to identify anomalies.
4.2 Correlate Threat Data
Use correlation engines in tools such as Darktrace to identify relationships between disparate threat indicators.
5. Reporting and Visualization
5.1 Generate Threat Reports
Create comprehensive reports using AI-driven reporting tools such as Tableau to visualize threat landscapes and trends.
5.2 Share Intelligence
Disseminate findings through threat intelligence platforms like VirusTotal and Anomali for collaborative analysis.
6. Response and Mitigation
6.1 Automate Incident Response
Implement orchestration tools like Palo Alto Networks Cortex XSOAR to automate incident response actions based on AI recommendations.
6.2 Continuous Improvement
Regularly review and refine AI models and processes based on feedback and new threat data to enhance future threat intelligence efforts.
7. Review and Iterate
7.1 Conduct Post-Incident Reviews
Analyze the effectiveness of the threat intelligence process and identify areas for improvement.
7.2 Update AI Models
Continuously update machine learning models with new data to improve detection rates and reduce false positives.
Keyword: AI threat intelligence process