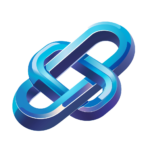
AI Integrated Weather Forecasting and Disaster Preparedness Solutions
AI-driven weather forecasting enhances disaster preparedness by integrating real-time meteorological and agricultural data for effective risk assessment and response planning.
Category: AI Security Tools
Industry: Agriculture
AI-Enabled Weather Forecasting and Disaster Preparedness
1. Data Collection
1.1 Meteorological Data
Utilize AI-driven tools to gather real-time meteorological data from various sources including satellite imagery, weather stations, and IoT devices.
1.2 Agricultural Data
Collect data on soil conditions, crop health, and historical yield patterns using sensors and drones equipped with AI capabilities.
2. Data Processing
2.1 Data Integration
Integrate meteorological and agricultural data using AI algorithms to create a comprehensive dataset for analysis.
2.2 Data Analysis
Implement machine learning models to analyze the integrated data, identifying patterns and predicting weather impacts on agriculture.
Example Tools:
- IBM Watson for Weather
- Climacell
3. Forecasting
3.1 Predictive Modeling
Develop predictive models using AI to forecast weather events and their potential impact on agricultural operations.
3.2 Scenario Simulation
Use AI simulations to model various disaster scenarios, helping farmers prepare for extreme weather conditions.
Example Tools:
- Google Cloud AI
- Microsoft Azure Machine Learning
4. Risk Assessment
4.1 Vulnerability Analysis
Employ AI tools to assess the vulnerability of crops and farming practices to predicted weather events.
4.2 Impact Evaluation
Utilize AI to evaluate the potential economic impact of adverse weather conditions on agricultural productivity.
Example Tools:
- Risk Management Solutions (RMS)
- AgriWebb
5. Disaster Preparedness Planning
5.1 Action Plan Development
Create actionable disaster response plans based on AI-generated forecasts and risk assessments.
5.2 Resource Allocation
Leverage AI to optimize the allocation of resources, such as irrigation systems and crop protection measures, in preparation for predicted weather events.
Example Tools:
- FarmLogs
- FieldView
6. Monitoring and Response
6.1 Real-Time Monitoring
Implement AI-driven monitoring systems to provide real-time updates on weather conditions and crop status during adverse events.
6.2 Adaptive Response
Utilize AI to adapt farming practices in real-time based on evolving weather conditions and forecasts.
Example Tools:
- Climate FieldView
- AgFunder Network Partners
7. Post-Disaster Analysis
7.1 Damage Assessment
Use AI tools to assess the extent of damage to crops and infrastructure following a weather event.
7.2 Lessons Learned
Analyze the effectiveness of disaster preparedness plans and make improvements for future events using AI-driven insights.
Example Tools:
- Farmers Edge
- AgriAnalytics
Keyword: AI weather forecasting for agriculture