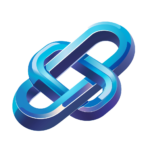
Enhancing Quality Control with AI Integration Workflow
Discover an AI-driven quality control enhancement pipeline that optimizes manufacturing processes through real-time data analysis and continuous improvement strategies
Category: AI Self Improvement Tools
Industry: Manufacturing and Industrial Automation
Quality Control Enhancement Pipeline
1. Initial Assessment
1.1 Define Quality Standards
Establish the quality benchmarks that products must meet based on industry regulations and customer expectations.
1.2 Identify Current Processes
Analyze existing quality control processes to identify strengths, weaknesses, and areas for improvement.
2. Data Collection
2.1 Implement Sensors and IoT Devices
Utilize Internet of Things (IoT) devices to gather real-time data from manufacturing equipment and production lines.
2.2 Integrate AI-Driven Data Analytics Tools
Deploy AI tools such as IBM Watson or Microsoft Azure Machine Learning to process and analyze collected data for insights.
3. AI Model Development
3.1 Select Machine Learning Algorithms
Choose appropriate machine learning algorithms (e.g., regression analysis, neural networks) to model quality outcomes based on historical data.
3.2 Train AI Models
Utilize training datasets to enhance the AI’s predictive capabilities regarding quality control issues.
4. Implementation of AI Solutions
4.1 Deploy AI-Driven Quality Control Systems
Integrate AI solutions such as Siemens’ MindSphere or GE’s Predix into the production workflow to automate quality checks.
4.2 Real-Time Monitoring and Alerts
Set up real-time monitoring systems to provide alerts for anomalies detected by AI, enabling immediate corrective actions.
5. Continuous Improvement
5.1 Feedback Loop Creation
Establish a feedback mechanism where data from quality control outcomes informs further refinements in AI models.
5.2 Regular Model Updates
Continuously update AI models based on new data and insights to enhance accuracy and reliability in quality assessments.
6. Reporting and Documentation
6.1 Generate Quality Reports
Utilize reporting tools such as Tableau or Power BI to visualize quality control data and trends for stakeholders.
6.2 Maintain Compliance Documentation
Ensure all quality control processes and outcomes are documented to meet regulatory compliance and internal audit requirements.
7. Training and Development
7.1 Employee Training Programs
Implement training sessions for employees on the use of AI tools and the importance of quality control in manufacturing processes.
7.2 Continuous Learning Initiatives
Encourage ongoing education on advancements in AI technologies and quality control methodologies.
Keyword: AI driven quality control solutions