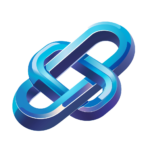
Refining Personalized Recommendation Engines with AI Integration
Discover how to refine a personalized recommendation engine using AI-driven workflows to enhance user engagement in the hospitality and tourism sectors.
Category: AI Self Improvement Tools
Industry: Hospitality and Tourism
Personalized Recommendation Engine Refinement
1. Define Objectives
1.1 Identify Key Performance Indicators (KPIs)
Establish measurable outcomes to evaluate the effectiveness of the recommendation engine.
1.2 Understand User Needs
Conduct surveys and interviews with hospitality and tourism customers to gather insights on their preferences and expectations.
2. Data Collection
2.1 Gather Historical Data
Collect historical booking data, user interactions, and feedback to inform the recommendation algorithms.
2.2 Integrate External Data Sources
Utilize APIs to incorporate data from social media, travel blogs, and review platforms to enhance contextual understanding.
3. AI Model Development
3.1 Select AI Techniques
Choose appropriate machine learning algorithms such as collaborative filtering, content-based filtering, or hybrid models.
3.2 Implement Natural Language Processing (NLP)
Utilize NLP tools like Google’s BERT or OpenAI’s GPT to analyze user-generated content and sentiment.
4. Tool Selection
4.1 Choose AI-Driven Products
Consider platforms such as:
- Salesforce Einstein: For predictive analytics and personalized marketing.
- IBM Watson: To enhance customer interactions through AI-driven chatbots.
- Revinate: For reputation management and customer feedback analysis.
4.2 Integrate with Existing Systems
Ensure compatibility with property management systems (PMS) and customer relationship management (CRM) tools.
5. Testing and Validation
5.1 A/B Testing
Conduct A/B tests to compare different recommendation strategies and their impact on user engagement.
5.2 User Feedback Loop
Establish mechanisms for continuous user feedback to refine recommendations based on real-time data.
6. Deployment
6.1 Implement the Recommendation Engine
Launch the refined recommendation engine on digital platforms such as websites and mobile applications.
6.2 Monitor Performance
Regularly track KPIs and user engagement metrics to assess the effectiveness of the recommendations.
7. Continuous Improvement
7.1 Iterate Based on Insights
Utilize analytics to make data-driven adjustments to the recommendation algorithms.
7.2 Stay Updated with AI Trends
Continuously research advancements in AI and machine learning to enhance the recommendation engine capabilities.
Keyword: personalized recommendation engine