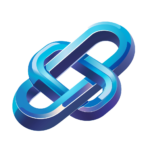
AI Driven Injury Risk Prediction and Prevention Workflow
AI-driven injury risk prediction and prevention enhances athlete safety through data collection analysis and personalized training strategies for optimal performance
Category: AI Sports Tools
Industry: Sports Scouting and Recruitment
Injury Risk Prediction and Prevention
1. Data Collection
1.1 Athlete Data Acquisition
Collect comprehensive data on athletes, including physical attributes, injury history, training loads, and performance metrics.
1.2 Wearable Technology Integration
Utilize wearable devices such as GPS trackers and heart rate monitors to gather real-time data on athlete performance and physiological responses during training and competition.
2. Data Processing and Analysis
2.1 Data Cleaning and Preparation
Ensure the data collected is clean, standardized, and ready for analysis. This includes removing duplicates and addressing missing values.
2.2 AI-Driven Analytics Tools
Implement AI-driven analytics platforms such as IBM Watson or Google Cloud AI to analyze patterns in the data. These tools can identify correlations between training loads and injury occurrences.
3. Injury Risk Assessment
3.1 Predictive Modeling
Develop predictive models using machine learning algorithms to assess the risk of injury. Tools like TensorFlow or PyTorch can be employed to create these models based on historical data.
3.2 Risk Scoring
Generate risk scores for individual athletes based on predictive analytics, highlighting those at higher risk for injury.
4. Prevention Strategies
4.1 Personalized Training Programs
Utilize insights from AI models to design personalized training regimens that mitigate injury risks, taking into account each athlete’s specific needs and risk factors.
4.2 Monitoring and Feedback Tools
Implement platforms like Kitman Labs or Catapult Sports that offer ongoing monitoring and feedback to coaches and athletes, allowing for real-time adjustments in training.
5. Continuous Evaluation and Improvement
5.1 Performance Tracking
Continuously track athlete performance and health metrics using AI tools to evaluate the effectiveness of prevention strategies.
5.2 Iterative Model Refinement
Regularly update predictive models with new data to enhance accuracy and adapt to changing athlete conditions and training environments.
6. Reporting and Communication
6.1 Stakeholder Reporting
Generate detailed reports for coaches, sports scientists, and management that summarize findings, risk assessments, and recommendations for injury prevention.
6.2 Athlete Education
Communicate findings to athletes to foster awareness and understanding of their injury risks and the importance of adherence to personalized training programs.
Keyword: Injury risk prediction strategies