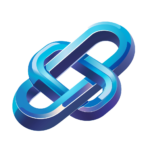
AI Driven Predictive Maintenance Workflow for Aircraft Efficiency
Discover AI-driven predictive maintenance for aircraft optimizing data collection processing and scheduling to enhance performance and reduce downtime
Category: AI Travel Tools
Industry: Airlines
Predictive Maintenance for Aircraft
1. Data Collection
1.1 Sensor Data Acquisition
Utilize IoT sensors installed on aircraft to continuously gather data on engine performance, temperature, vibration, and fuel consumption.
1.2 Historical Maintenance Records
Compile historical maintenance data, including past repairs, replacements, and service schedules, to establish a baseline for predictive analysis.
2. Data Processing
2.1 Data Integration
Integrate data from various sources, including sensor data, maintenance logs, and flight data, into a centralized database for analysis.
2.2 Data Cleaning
Utilize AI-driven tools such as IBM Watson or Google Cloud AI to clean and preprocess the data, ensuring accuracy and consistency.
3. Predictive Analytics
3.1 Machine Learning Model Development
Develop machine learning models using platforms like Microsoft Azure Machine Learning or TensorFlow to predict potential failures based on historical and real-time data.
3.2 Risk Assessment
Implement AI algorithms to assess the risk of component failures, categorizing them by severity and likelihood, enabling prioritization of maintenance tasks.
4. Maintenance Scheduling
4.1 Automated Scheduling
Use AI-driven scheduling tools such as Airline Maintenance Management Systems (AMMS) to automate maintenance scheduling based on predictive analytics results.
4.2 Resource Allocation
Leverage AI to optimize resource allocation, ensuring that the right personnel and parts are available for scheduled maintenance.
5. Continuous Monitoring
5.1 Real-Time Monitoring Tools
Implement real-time monitoring solutions such as GE Aviation’s Digital Wind Farm to continuously track aircraft performance and predict maintenance needs dynamically.
5.2 Feedback Loop
Create a feedback loop where data from completed maintenance activities is fed back into the system to improve the accuracy of predictive models.
6. Reporting and Analysis
6.1 Performance Metrics
Generate reports using AI-powered analytics tools to evaluate maintenance effectiveness, downtime, and cost savings achieved through predictive maintenance.
6.2 Strategic Decision Making
Utilize insights from data analysis to inform strategic decisions regarding fleet management, operational efficiency, and investment in new technologies.
7. Continuous Improvement
7.1 Model Refinement
Regularly refine machine learning models based on new data and outcomes to enhance predictive accuracy and reliability.
7.2 Technology Upgrades
Stay updated with advancements in AI and machine learning technologies, integrating new tools and methodologies to continuously improve the predictive maintenance process.
Keyword: Predictive maintenance for aircraft