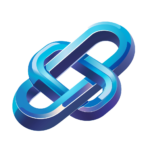
AI Driven Predictive Analytics for Hurricane Path Forecasting
AI-driven predictive analytics enhances hurricane path forecasting and preparedness through data collection model development and effective communication strategies
Category: AI Weather Tools
Industry: Emergency Services
Predictive Analytics for Hurricane Path Forecasting and Preparedness
1. Data Collection
1.1. Meteorological Data
Gather historical and real-time meteorological data from various sources, including:
- National Oceanic and Atmospheric Administration (NOAA)
- Satellite imagery
- Weather stations
1.2. Social and Infrastructure Data
Compile data on population density, infrastructure, and emergency resources:
- Demographic information
- Transportation networks
- Emergency service locations
2. Data Preprocessing
2.1. Data Cleaning
Ensure data accuracy by removing outliers and correcting inconsistencies.
2.2. Data Normalization
Standardize data formats to facilitate analysis.
3. AI Model Development
3.1. Selection of AI Tools
Utilize AI-driven products such as:
- TensorFlow: For building machine learning models.
- IBM Watson: For natural language processing and predictive analytics.
- Microsoft Azure Machine Learning: For deploying AI models at scale.
3.2. Model Training
Train machine learning models using historical data to predict hurricane paths, employing algorithms such as:
- Random Forest
- Neural Networks
4. Predictive Analytics
4.1. Path Forecasting
Utilize trained models to forecast hurricane paths and intensity, generating visualizations and reports.
4.2. Scenario Analysis
Conduct simulations to assess potential impacts under various scenarios, incorporating variables such as:
- Wind speed
- Storm surge
5. Decision Support
5.1. Risk Assessment
Evaluate risks to populations and infrastructure based on predictive analytics outcomes.
5.2. Resource Allocation
Utilize AI tools to optimize resource deployment, ensuring emergency services are prepared and positioned effectively.
6. Communication and Reporting
6.1. Stakeholder Engagement
Share findings with relevant stakeholders, including:
- Emergency management agencies
- Local government officials
6.2. Public Awareness
Disseminate information to the public through various channels, including:
- Social media
- Mobile alerts
7. Continuous Improvement
7.1. Feedback Loop
Collect feedback from stakeholders and the public to refine predictive models.
7.2. Model Updates
Regularly update AI models with new data to enhance forecasting accuracy and responsiveness.
Keyword: AI hurricane path forecasting