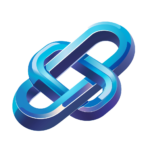
AI Integration in Water Quality Management for Weather Changes
AI-driven water quality management leverages real-time weather data and IoT sensors to optimize aquatic health and support sustainable fishing practices
Category: AI Weather Tools
Industry: Fishing and Aquaculture
AI-Driven Water Quality Management in Changing Weather
1. Data Collection
1.1 Weather Data Acquisition
Utilize AI-driven weather forecasting tools to gather real-time and predictive weather data. Tools such as IBM’s The Weather Company and AccuWeather can provide hyper-local forecasts.
1.2 Water Quality Monitoring
Deploy IoT sensors to monitor key water quality parameters, including pH, temperature, dissolved oxygen, and turbidity. Examples include YSI ProDSS and AquaTroll 600.
2. Data Integration
2.1 Centralized Data Management
Implement a cloud-based data management system to integrate weather data and water quality metrics. Platforms like Microsoft Azure or Google Cloud can facilitate this integration.
2.2 AI Model Training
Utilize machine learning algorithms to analyze historical data and train models for predicting water quality changes due to weather variations. Tools such as TensorFlow or Scikit-learn can be employed for this purpose.
3. Predictive Analysis
3.1 Risk Assessment
Conduct risk assessments based on predictive models to identify potential adverse impacts on aquatic life and fishing activities. AI tools like RapidMiner can assist in generating risk profiles.
3.2 Decision Support Systems
Develop AI-driven decision support systems that provide actionable insights for fishers and aquaculture operators. Systems like IBM Watson can analyze data and suggest optimal fishing times and locations.
4. Implementation of Recommendations
4.1 Adaptive Management Strategies
Implement adaptive management strategies based on AI recommendations to mitigate risks. This may include adjusting feeding schedules or changing fishing techniques.
4.2 Continuous Monitoring and Feedback Loop
Establish a feedback loop where ongoing monitoring informs future AI model adjustments. Utilize platforms like Tableau for real-time data visualization and reporting.
5. Stakeholder Engagement
5.1 Training and Development
Provide training sessions for stakeholders on the use of AI tools and the interpretation of data insights. Workshops can be facilitated through platforms like Zoom or Microsoft Teams.
5.2 Community Collaboration
Engage with local fishing communities and aquaculture operators to share insights and best practices. Utilize collaborative platforms such as Slack or Asana for ongoing communication.
6. Review and Optimization
6.1 Performance Evaluation
Regularly evaluate the performance of AI models and the effectiveness of implemented strategies. Utilize KPIs and metrics to assess outcomes.
6.2 Continuous Improvement
Incorporate findings from performance evaluations into the AI models to enhance predictive accuracy and operational efficiency. Schedule regular review meetings for strategy optimization.
Keyword: AI water quality management system