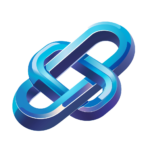
AI Content Recommendation Engine Workflow for Enhanced Engagement
AI-powered content recommendation engine enhances user engagement through data-driven insights and personalized suggestions for optimal content discovery
Category: AI Website Tools
Industry: Media and Entertainment
AI-Powered Content Recommendation Engine Implementation
1. Project Initiation
1.1 Define Objectives
Establish clear goals for the content recommendation engine, focusing on enhancing user engagement and content discovery.
1.2 Assemble Project Team
Gather a cross-functional team including data scientists, software developers, and content strategists.
2. Research and Selection of AI Tools
2.1 Identify Requirements
Determine the technical and functional requirements for the recommendation engine.
2.2 Evaluate AI Tools
Research and compare various AI-driven products such as:
- Google Cloud AI: Offers machine learning capabilities for personalized content recommendations.
- Amazon Personalize: A service that allows developers to create individualized recommendations using machine learning.
- IBM Watson: Provides AI tools for analyzing user behavior and preferences.
2.3 Select Tools
Choose the most suitable AI tools based on the evaluation criteria established in the previous step.
3. Data Collection and Preparation
3.1 Gather User Data
Collect user interaction data, including clicks, views, and engagement metrics from existing platforms.
3.2 Clean and Organize Data
Ensure data quality by cleaning and organizing the collected data for analysis.
4. Model Development
4.1 Choose Recommendation Algorithm
Select an appropriate algorithm, such as collaborative filtering or content-based filtering, based on project objectives.
4.2 Train AI Model
Utilize the selected AI tools to train the recommendation model using the prepared data.
5. Testing and Validation
5.1 Conduct A/B Testing
Implement A/B testing to evaluate the effectiveness of the recommendation engine against existing content delivery methods.
5.2 Analyze Results
Assess user engagement metrics and feedback to validate the performance of the recommendation engine.
6. Implementation
6.1 Integrate with Existing Systems
Work with IT teams to integrate the recommendation engine into current website infrastructure.
6.2 Launch Recommendation Engine
Officially launch the AI-powered content recommendation engine to users.
7. Monitoring and Optimization
7.1 Monitor Performance
Continuously track the performance of the recommendation engine using key performance indicators (KPIs).
7.2 Optimize Algorithms
Regularly update and refine algorithms based on user feedback and changing content trends.
8. Reporting and Review
8.1 Generate Reports
Compile reports on user engagement, satisfaction, and overall performance of the recommendation engine.
8.2 Conduct Project Review
Hold a review meeting with stakeholders to discuss outcomes, lessons learned, and future enhancements.
Keyword: AI content recommendation engine