Streamline Inventory Management with AI for Developers Guide
Topic: AI Developer Tools
Industry: Logistics and Supply Chain
Discover how AI transforms inventory management with predictive analytics automated replenishment and real-time tracking in this essential developer’s guide
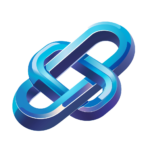
Streamlining Inventory Management with AI: A Developer’s Guide
Understanding the Role of AI in Inventory Management
Artificial Intelligence (AI) has emerged as a transformative force in various industries, particularly in logistics and supply chain management. By automating processes, enhancing decision-making, and providing predictive analytics, AI can significantly streamline inventory management. For developers looking to leverage AI in this domain, understanding the available tools and methodologies is crucial.Key Components of AI-Driven Inventory Management
To effectively streamline inventory management, developers should focus on several key components:1. Predictive Analytics
Predictive analytics involves using historical data to forecast future inventory needs. This can help businesses maintain optimal stock levels, reduce excess inventory, and minimize stockouts. Tools such as Microsoft Azure Machine Learning and Google Cloud AI offer robust platforms for building predictive models that can analyze trends and patterns in inventory data.2. Automated Replenishment
Automated replenishment systems utilize AI algorithms to determine when to reorder stock based on demand forecasts. Solutions like Oracle NetSuite and SAP Integrated Business Planning provide features that automate the replenishment process, ensuring that businesses maintain optimal inventory levels without manual intervention.3. Real-Time Inventory Tracking
Real-time inventory tracking is essential for maintaining visibility across the supply chain. AI-driven tools such as RFID technology and IoT sensors can provide real-time data on inventory levels. Companies can implement solutions like IBM Watson IoT to integrate these technologies, allowing for seamless tracking and management of inventory.4. Demand Forecasting
Accurate demand forecasting is critical for effective inventory management. AI can analyze various factors, such as seasonality, market trends, and consumer behavior, to predict future demand. Tools like Forecast Pro and Demand Works provide AI-driven forecasting capabilities that can enhance decision-making processes.Implementing AI Solutions in Inventory Management
To implement AI solutions effectively, developers should consider the following steps:1. Assess Current Inventory Management Processes
Before integrating AI, it is essential to evaluate existing inventory management processes. Identify pain points, inefficiencies, and areas where AI can add value. This assessment will guide the selection of appropriate AI tools and technologies.2. Choose the Right AI Tools
Selecting the right AI tools is crucial for successful implementation. Developers should consider factors such as scalability, ease of integration, and the specific needs of the business. Popular AI tools for inventory management include:- Zoho Inventory: An AI-powered inventory management software that automates stock tracking and order management.
- Clear Spider: A cloud-based inventory management system that utilizes AI for real-time tracking and analytics.
- Fishbowl: An inventory management solution that integrates with various e-commerce platforms and uses AI for demand forecasting.
3. Train and Optimize AI Models
Once the tools are in place, it is essential to train and optimize AI models using historical data. This process involves feeding the AI algorithms with relevant data and continuously refining them to improve accuracy over time.4. Monitor and Evaluate Performance
After implementation, monitoring the performance of AI solutions is vital. Collect data on key performance indicators (KPIs) such as inventory turnover rates, stockout occurrences, and overall operational efficiency. This data will help assess the effectiveness of the AI tools and inform future adjustments.Conclusion
The integration of AI in inventory management can lead to significant efficiencies and cost savings for businesses in the logistics and supply chain sector. By leveraging predictive analytics, automated replenishment, real-time tracking, and demand forecasting, developers can create robust AI-driven solutions that enhance inventory management processes. As technology continues to evolve, staying informed about the latest AI tools and methodologies will be essential for developers aiming to drive innovation in this critical area of business.Keyword: AI inventory management solutions