The Future of Personalized Streaming with AI Recommendation Engines
Topic: AI Domain Tools
Industry: Media and Entertainment
Discover how AI recommendation engines are shaping the future of personalized streaming by enhancing user engagement and delivering tailored content experiences.
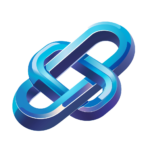
The Future of Personalized Streaming: AI Recommendation Engines Explained
Understanding AI Recommendation Engines
Artificial intelligence (AI) has revolutionized various sectors, and the media and entertainment industry is no exception. At the heart of this transformation are AI recommendation engines, which analyze user behavior and preferences to deliver personalized content. These engines leverage complex algorithms and machine learning techniques to curate experiences that resonate with individual users, thereby enhancing viewer satisfaction and engagement.The Role of AI in Media and Entertainment
In the context of media and entertainment, AI can be implemented in numerous ways. By analyzing vast amounts of data, AI systems can identify patterns in user behavior, such as viewing habits, genre preferences, and even the time spent on specific types of content. This information can then be used to generate tailored recommendations, ensuring that users are presented with content that aligns with their interests.Key Components of AI Recommendation Engines
1. Data Collection: The first step involves gathering data from various sources, including user interactions, social media activity, and demographic information. Tools like Google Analytics and Mixpanel can be instrumental in this phase, providing insights into user engagement. 2. Data Processing: Once the data is collected, it is processed using machine learning algorithms. These algorithms can be supervised or unsupervised, depending on the nature of the data and the desired outcomes. Frameworks such as TensorFlow and PyTorch are commonly used for building these models. 3. Recommendation Algorithms: The next step is the application of recommendation algorithms, which can be categorized into collaborative filtering, content-based filtering, and hybrid methods. Collaborative filtering analyzes user behavior to find similarities among users, while content-based filtering focuses on the attributes of the items themselves. Hybrid methods combine both approaches for enhanced accuracy. 4. Continuous Learning: AI recommendation engines are designed to learn continuously. As users interact with the platform, the system adapts and refines its recommendations. This is where tools like Amazon Personalize come into play, allowing businesses to implement machine learning models that evolve based on user feedback.Examples of AI-Driven Products in Streaming
Several companies have successfully integrated AI-driven recommendation engines into their streaming services, demonstrating the potential of this technology.1. Netflix
Netflix is a prime example of effective AI implementation in streaming. The platform uses sophisticated algorithms to analyze user viewing patterns and preferences. By employing collaborative filtering and deep learning techniques, Netflix can suggest shows and movies that align with individual tastes, significantly enhancing user engagement.2. Spotify
Spotify revolutionized music streaming by utilizing AI to create personalized playlists, such as “Discover Weekly.” The platform analyzes listening habits and preferences to curate a unique selection of songs for each user. This level of personalization not only keeps users engaged but also fosters loyalty to the platform.3. YouTube
YouTube’s recommendation system is another exemplary model of AI in action. By analyzing user interaction data, YouTube can recommend videos that users are likely to enjoy. The platform employs deep learning algorithms to predict user preferences, ensuring a tailored viewing experience.Challenges and Considerations
While the benefits of AI recommendation engines are substantial, there are challenges that need to be addressed. Data privacy is a significant concern, as users may be wary of how their information is collected and utilized. Companies must ensure compliance with data protection regulations and maintain transparency about their data practices. Additionally, the risk of creating echo chambers, where users are only exposed to content that reinforces their existing preferences, poses a challenge. Striking a balance between personalization and diversity in content recommendations is crucial for fostering a well-rounded viewing experience.Conclusion
The future of personalized streaming is undoubtedly intertwined with the advancements in AI recommendation engines. As media and entertainment companies continue to harness the power of artificial intelligence, the potential for delivering tailored content experiences will only grow. By understanding and implementing these technologies responsibly, businesses can enhance user engagement, drive satisfaction, and ultimately, foster loyalty in an increasingly competitive landscape.Keyword: personalized streaming recommendations