The Impact of NLP on Pharma Scientific Networking and Collaboration
Topic: AI Networking Tools
Industry: Pharmaceuticals and Biotechnology
Discover how Natural Language Processing is transforming scientific networking in pharma by enhancing collaboration communication and research efficiency.
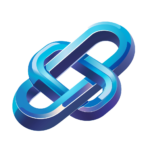
The Impact of Natural Language Processing on Scientific Networking in Pharma
Introduction to Natural Language Processing in Pharma
Natural Language Processing (NLP) is revolutionizing various sectors, and the pharmaceutical and biotechnology industries are no exception. As the complexity of scientific research increases, the need for efficient communication and data interpretation becomes paramount. NLP, a branch of artificial intelligence (AI), enables machines to understand, interpret, and generate human language, thus facilitating enhanced networking and collaboration among scientists, researchers, and healthcare professionals.
The Role of AI Networking Tools in Pharmaceuticals
AI networking tools are designed to streamline communication, enhance data sharing, and foster collaboration within the pharmaceutical sector. By leveraging NLP capabilities, these tools can analyze vast amounts of scientific literature, clinical trial data, and patient records to extract relevant insights. This not only accelerates the research process but also aids in the identification of potential collaborators and funding opportunities.
Key Benefits of NLP in Scientific Networking
- Improved Data Accessibility: NLP tools can sift through large datasets and scientific papers, making it easier for researchers to find pertinent information quickly.
- Enhanced Collaboration: By connecting researchers with similar interests and expertise, NLP tools foster collaborative projects that can lead to innovative solutions.
- Streamlined Communication: NLP facilitates clearer communication by summarizing complex information and providing insights in a user-friendly format.
Examples of AI-Driven Products and Tools
Several AI-driven products harness the power of NLP to improve scientific networking in the pharmaceutical industry. Here are a few noteworthy examples:
1. Elsevier’s PharmaPendium
PharmaPendium is a comprehensive database that provides access to FDA drug approval documents. Utilizing NLP, it allows researchers to quickly find relevant drug information, including clinical trial results and safety data. This tool enhances networking by enabling researchers to identify potential partners and competitors in drug development.
2. IBM Watson for Drug Discovery
IBM Watson for Drug Discovery employs NLP to analyze scientific literature and clinical trial data. By extracting insights from unstructured data, Watson helps researchers identify new drug candidates and potential therapeutic targets. This capability promotes collaboration among scientists by connecting them with relevant research and findings.
3. Bioz
Bioz is an AI-powered search engine that utilizes NLP to provide researchers with access to experimental protocols, reagents, and scientific literature. By streamlining the research process, Bioz enables scientists to identify collaborators and resources more effectively, enhancing networking opportunities within the biotech community.
Implementing NLP in Scientific Networking
For pharmaceutical companies looking to implement NLP-driven networking tools, the following strategies can be beneficial:
1. Invest in AI Training
Training staff on how to effectively use NLP tools can maximize their potential. This includes understanding how to interpret data outputs and leverage insights for strategic decision-making.
2. Foster an Open Collaboration Culture
Encouraging a culture of collaboration can enhance the effectiveness of NLP tools. By promoting open communication and knowledge sharing, organizations can create an environment where innovative ideas flourish.
3. Integrate with Existing Systems
To fully realize the benefits of NLP, companies should integrate these tools with existing research management systems. This ensures a seamless flow of information and enhances the overall research process.
Conclusion
The integration of Natural Language Processing into scientific networking is transforming the pharmaceutical and biotechnology industries. By leveraging AI-driven tools, organizations can enhance collaboration, streamline communication, and accelerate research efforts. As the landscape of drug development continues to evolve, embracing these technologies will be crucial for staying competitive and fostering innovation.
Keyword: natural language processing in pharma