The Rise of Federated Learning in Telecom AI Privacy Solutions
Topic: AI Privacy Tools
Industry: Telecommunications
Discover how federated learning enhances AI in telecommunications by preserving user privacy while optimizing services and ensuring compliance with regulations.
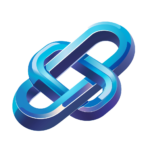
The Rise of Federated Learning: Preserving Privacy in Telecom AI Models
Understanding Federated Learning
Federated learning is an innovative approach to machine learning that enables multiple parties to collaboratively train models without sharing their raw data. This decentralized method is particularly beneficial in the telecommunications sector, where sensitive customer information must be protected. By allowing data to remain on local devices while only sharing model updates, federated learning ensures that privacy is maintained while still harnessing the power of artificial intelligence (AI).
The Importance of Privacy in Telecommunications
In an era where data breaches and privacy concerns are at the forefront of consumer awareness, telecommunications companies face the challenge of utilizing AI while safeguarding user information. With regulations such as the General Data Protection Regulation (GDPR) and the California Consumer Privacy Act (CCPA) imposing strict guidelines on data handling, the need for robust privacy-preserving technologies is paramount.
AI Applications in Telecommunications
Artificial intelligence can be implemented in various ways within the telecommunications industry, enhancing customer service, optimizing network performance, and improving predictive maintenance. However, traditional AI models often require access to large datasets, which can compromise user privacy. This is where federated learning comes into play, allowing telecom companies to develop AI models without compromising sensitive information.
Examples of AI-Driven Products Utilizing Federated Learning
Several AI-driven products have begun to incorporate federated learning, demonstrating its potential in the telecommunications space:
1. Customer Experience Enhancement Tools
Telecom companies can deploy AI-driven chatbots and virtual assistants that learn from user interactions while ensuring that individual conversations remain private. By using federated learning, these tools can improve their responses based on aggregated insights without accessing personal data.
2. Network Optimization Solutions
AI algorithms can analyze network performance data to predict and mitigate outages. With federated learning, telecom companies can train these models using data from various network nodes without exposing sensitive operational data. This leads to improved service reliability while maintaining user privacy.
3. Fraud Detection Systems
Fraud detection is critical in the telecommunications industry. By employing federated learning, companies can develop AI models that detect fraudulent activities based on patterns learned from distributed data sources. This approach minimizes the risk of exposing sensitive customer information during the training process.
Challenges and Considerations
While federated learning offers significant advantages, it is not without challenges. Ensuring the quality of model updates, managing communication overhead, and addressing potential biases in decentralized datasets are critical factors that need to be considered. Telecom companies must invest in robust infrastructure and expertise to effectively implement federated learning solutions.
The Future of AI in Telecommunications
As the telecommunications industry continues to evolve, the integration of AI and privacy-preserving technologies like federated learning will play a crucial role in shaping the future. By adopting these innovative approaches, telecom companies can enhance their services while building trust with their customers. The rise of federated learning marks a significant step towards achieving a balance between leveraging data for AI advancements and protecting user privacy.
Conclusion
In conclusion, the rise of federated learning represents a pivotal moment in the telecommunications sector’s approach to AI. By prioritizing privacy and utilizing advanced AI-driven tools, telecom companies can not only comply with regulatory requirements but also foster a more secure and trustworthy relationship with their customers. As this technology matures, it will undoubtedly pave the way for a new era of privacy-conscious AI applications in telecommunications.
Keyword: federated learning in telecommunications