Top 5 AI Coding Tools Transforming Biotech Research in 2025
Topic: AI Coding Tools
Industry: Biotechnology
Discover the top 5 AI coding tools transforming biotech research in 2025 from AlphaFold to Benchling and enhance your research efficiency today
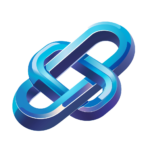
Top 5 AI Coding Tools Revolutionizing Biotech Research in 2025
Introduction
The biotechnology sector is experiencing a significant transformation, propelled by advancements in artificial intelligence (AI). As we move into 2025, AI coding tools are emerging as pivotal resources that enhance research efficiency, data analysis, and product development. This article explores five leading AI coding tools that are revolutionizing biotech research, showcasing how they can be effectively implemented in various applications.
1. DeepMind’s AlphaFold
AlphaFold has made headlines for its groundbreaking ability to predict protein structures with remarkable accuracy. This AI-driven tool utilizes deep learning techniques to analyze amino acid sequences and predict their three-dimensional configurations. In 2025, researchers can implement AlphaFold to expedite drug discovery processes by providing insights into protein interactions and facilitating the design of novel therapeutics.
Implementation Example
Biotech companies can integrate AlphaFold into their existing research pipelines to enhance structural biology studies. By inputting genetic sequences, researchers can receive rapid predictions, allowing them to focus on experimental validation and further exploration of protein functions.
2. IBM Watson for Drug Discovery
IBM Watson continues to be a leader in AI applications for healthcare and biotechnology. Its Drug Discovery platform leverages machine learning algorithms to analyze vast datasets, including scientific literature, clinical trial data, and patient records. In 2025, Watson’s capabilities will enable faster identification of potential drug candidates and biomarkers, streamlining the development process.
Implementation Example
Biotech firms can utilize Watson to conduct comprehensive literature reviews and extract relevant insights, significantly reducing the time spent on manual data analysis. By automating the identification of trends and correlations, Watson empowers researchers to make data-driven decisions more efficiently.
3. BioSymphony
BioSymphony is an innovative AI platform designed specifically for the biotechnology sector. It employs natural language processing (NLP) to facilitate the analysis of scientific texts and patents. In 2025, BioSymphony will be instrumental in helping researchers stay ahead of the curve by identifying emerging trends and technologies in biotech.
Implementation Example
Research institutions can deploy BioSymphony to monitor the latest publications and patent filings, ensuring that their teams are informed about cutting-edge developments. This tool can also assist in competitive analysis, helping organizations strategize their research focus based on current market trends.
4. LabArchives
LabArchives is a cloud-based electronic lab notebook (ELN) that incorporates AI functionalities to enhance data management and collaboration among researchers. In 2025, this tool will play a crucial role in integrating AI-driven analytics with experimental data, providing real-time insights and predictive modeling capabilities.
Implementation Example
Biotech labs can implement LabArchives to streamline their documentation processes while leveraging AI to analyze experimental results. By utilizing its analytics features, researchers can uncover patterns in their data, leading to more informed experimental designs and faster iterations.
5. Benchling
Benchling is a comprehensive life sciences R&D cloud platform that integrates AI tools to enhance workflow efficiency. In 2025, Benchling’s AI capabilities will allow researchers to automate routine tasks, such as data entry and analysis, enabling them to focus more on innovative research.
Implementation Example
Organizations can adopt Benchling to unify their research efforts across various teams. By utilizing its AI-driven automation features, researchers can ensure that data integrity is maintained while accelerating the pace of discovery through collaborative efforts.
Conclusion
The integration of AI coding tools into biotechnology research is not merely a trend but a necessity for staying competitive in a rapidly evolving field. As we look ahead to 2025, tools like AlphaFold, IBM Watson, BioSymphony, LabArchives, and Benchling will continue to redefine how research is conducted, driving innovation and efficiency. Embracing these technologies will empower biotech organizations to overcome challenges and unlock new possibilities in drug discovery and development.
Keyword: AI coding tools for biotech research