Transforming Factory Operations with Predictive Maintenance AI
Topic: AI Research Tools
Industry: Manufacturing
Discover how predictive maintenance AI is revolutionizing factory operations by reducing downtime and costs while enhancing efficiency and safety in manufacturing
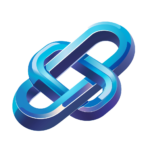
How Predictive Maintenance AI is Transforming Factory Operations
The Role of Predictive Maintenance in Manufacturing
In the fast-paced world of manufacturing, the need for efficiency and reliability has never been greater. Predictive maintenance (PdM) has emerged as a critical component in optimizing factory operations. By leveraging artificial intelligence (AI), manufacturers can anticipate equipment failures before they occur, thereby minimizing downtime and reducing maintenance costs.
Understanding Predictive Maintenance AI
Predictive maintenance AI utilizes machine learning algorithms and data analytics to monitor the condition of equipment in real-time. By analyzing historical data and current performance metrics, these systems can identify patterns that indicate potential failures. This proactive approach not only enhances operational efficiency but also extends the lifespan of machinery.
Key Benefits of Implementing Predictive Maintenance AI
- Reduced Downtime: By predicting equipment failures, manufacturers can schedule maintenance during non-productive hours, significantly reducing unexpected downtime.
- Cost Savings: Early detection of issues allows for timely repairs, preventing costly breakdowns and extending the life of machinery.
- Improved Safety: Predictive maintenance contributes to a safer work environment by identifying potential hazards before they result in accidents.
- Data-Driven Decisions: The insights gained from predictive maintenance AI empower manufacturers to make informed decisions about asset management and resource allocation.
Implementing Predictive Maintenance AI in Manufacturing
To successfully implement predictive maintenance AI, manufacturers must follow a structured approach that includes data collection, analysis, and integration with existing systems. Here are some steps to consider:
1. Data Collection
The first step involves gathering data from various sources, including sensors, equipment logs, and maintenance records. This data serves as the foundation for predictive analytics.
2. Choosing the Right Tools
Several AI-driven tools can facilitate predictive maintenance in manufacturing. Some notable examples include:
- Predictive Analytics Software: Tools like IBM Watson IoT and Siemens MindSphere provide advanced analytics capabilities, enabling manufacturers to analyze vast amounts of data and derive actionable insights.
- Condition Monitoring Sensors: Devices such as vibration sensors and thermal cameras can continuously monitor equipment health, feeding real-time data into predictive maintenance systems.
- Machine Learning Platforms: Platforms like Google Cloud AI and Microsoft Azure Machine Learning offer machine learning algorithms that can be tailored to specific manufacturing needs, enhancing predictive capabilities.
3. Integration with Existing Systems
For predictive maintenance AI to be effective, it must be integrated with existing manufacturing systems, such as Enterprise Resource Planning (ERP) and Manufacturing Execution Systems (MES). This integration ensures that insights from predictive maintenance are accessible across the organization, enabling a holistic approach to operations management.
Case Studies: Successful Implementation of Predictive Maintenance AI
Example 1: General Electric (GE)
General Electric has successfully implemented predictive maintenance AI across its manufacturing facilities. By utilizing advanced analytics and IoT sensors, GE has reduced unplanned downtime by 10-20%, resulting in significant cost savings and improved operational efficiency.
Example 2: Bosch
Bosch has integrated predictive maintenance AI into its manufacturing processes, utilizing machine learning algorithms to analyze data from its production lines. This initiative has led to a 25% reduction in maintenance costs and improved product quality through timely interventions.
Conclusion
The integration of predictive maintenance AI into factory operations is not just a trend; it is a necessity for manufacturers looking to stay competitive in an increasingly complex landscape. By harnessing the power of AI, organizations can optimize their maintenance strategies, reduce costs, and enhance overall productivity. As technology continues to evolve, the potential for predictive maintenance AI will only grow, paving the way for smarter and more efficient manufacturing processes.
Keyword: Predictive maintenance AI benefits