Transforming Vehicle Maintenance with Predictive Analytics
Topic: AI Data Tools
Industry: Automotive
Discover how predictive analytics is transforming vehicle maintenance by reducing downtime enhancing safety and optimizing costs for the automotive industry.
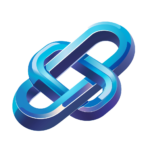
How Predictive Analytics is Transforming Vehicle Maintenance and Reducing Downtime
The Rise of Predictive Analytics in Automotive Maintenance
In recent years, the automotive industry has witnessed a significant transformation driven by advancements in predictive analytics. This data-driven approach is reshaping how vehicle maintenance is performed, enabling organizations to proactively address issues before they escalate into costly repairs or extended downtimes. By leveraging artificial intelligence (AI) and sophisticated data tools, automotive companies can enhance their maintenance strategies, improve operational efficiency, and ultimately deliver better service to their customers.Understanding Predictive Analytics
Predictive analytics involves using statistical algorithms and machine learning techniques to identify patterns in historical data. In the context of vehicle maintenance, this means analyzing various data points—such as vehicle performance metrics, sensor readings, and maintenance history—to forecast potential failures and maintenance needs. By predicting when a vehicle is likely to require servicing, businesses can schedule maintenance more effectively, reducing unexpected breakdowns and optimizing resource allocation.Key Benefits of Predictive Analytics in Vehicle Maintenance
1. Reduced Downtime: By anticipating maintenance needs, companies can minimize unplanned vehicle downtimes, ensuring that fleets remain operational and productive. 2. Cost Savings: Predictive maintenance can lead to significant cost reductions by preventing major repairs and extending the lifespan of vehicle components. 3. Enhanced Safety: Regularly maintained vehicles are safer on the road, reducing the risk of accidents caused by mechanical failures. 4. Data-Driven Decision Making: Access to real-time data allows fleet managers to make informed decisions about vehicle usage and maintenance schedules.Implementing AI in Predictive Maintenance
To harness the full potential of predictive analytics, automotive companies must integrate AI-driven tools into their operations. Here are several examples of how AI can be implemented in this context:1. IoT Sensors and Data Collection
The Internet of Things (IoT) plays a crucial role in predictive maintenance by enabling the collection of real-time data from vehicles. Sensors installed in various components can monitor metrics such as engine temperature, oil pressure, and tire health. Tools like IBM Watson IoT allow businesses to analyze this data, providing insights into vehicle performance and predicting maintenance needs.2. Machine Learning Algorithms
Machine learning algorithms can process vast amounts of data to identify patterns that might not be immediately obvious. For instance, SAS Predictive Analytics offers machine learning capabilities that can analyze historical maintenance data to predict future failures. This allows companies to implement maintenance schedules based on actual vehicle conditions rather than arbitrary time intervals.3. Fleet Management Software
Comprehensive fleet management solutions like Teletrac Navman and Geotab incorporate predictive analytics features that help fleet managers monitor vehicle health and performance. These platforms can alert managers to potential issues, enabling timely interventions that prevent breakdowns and reduce downtime.4. AI-Driven Diagnostic Tools
AI-driven diagnostic tools, such as Carmudi and AutonomouStuff, use advanced algorithms to analyze vehicle data and provide insights into potential mechanical issues. These tools can facilitate quicker diagnostics, allowing technicians to address problems more efficiently.Case Studies: Success Stories in Predictive Maintenance
Several companies have successfully implemented predictive analytics to enhance their vehicle maintenance processes.1. UPS
UPS has adopted predictive analytics to monitor its fleet of delivery vehicles. By analyzing data from sensors and historical maintenance records, UPS can predict when vehicles require servicing, resulting in reduced downtime and improved delivery efficiency.2. Tesla
Tesla utilizes predictive analytics to monitor the performance of its electric vehicles. The data collected from its fleet enables the company to identify potential issues and notify owners before they become critical, enhancing customer satisfaction and vehicle reliability.Conclusion
Predictive analytics is revolutionizing vehicle maintenance, offering automotive companies the tools they need to reduce downtime and improve operational efficiency. By implementing AI-driven solutions, organizations can not only enhance their maintenance strategies but also deliver superior service to their customers. As the technology continues to evolve, the potential for predictive analytics in the automotive sector will only grow, paving the way for smarter, more efficient vehicle management practices.Keyword: Predictive analytics vehicle maintenance